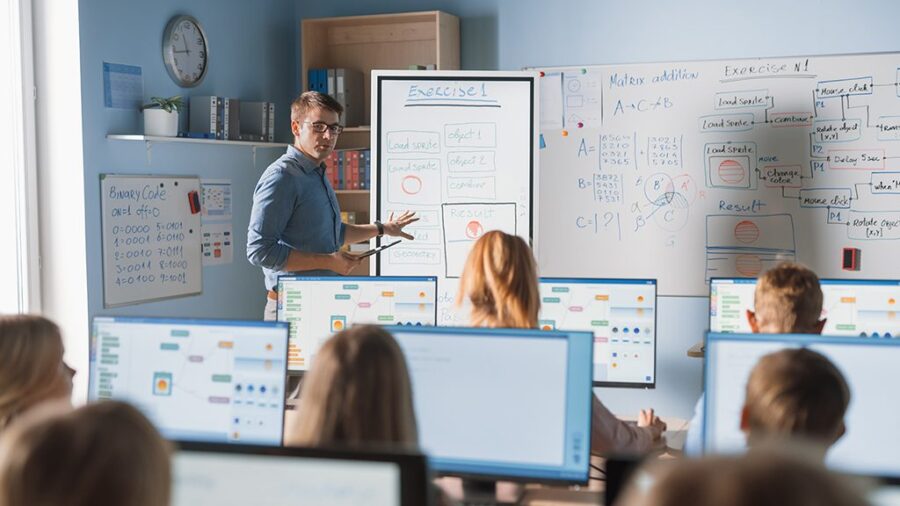
AI is rapidly changing the field of education. The advances in the data storage system and advancements made in Artificial Intelligence (AI) in the last decade have allowed organizations to store huge amounts of data and utilize that data to produce prescriptive insights.
The educational sector has also witnessed positive changes in the last few years. Electronic versions have replaced print-based books. Massive online courses appeared, and the advent of smaller learning units is eliminating lengthy lectures.
The innovations in the educational sector adapting to the changing preferences of the millennials and generation-Z’s have transformed the education sector in the way we think of it. The Covid-19 pandemic has led to specific changes that are now happening in the field of education.
How the Shift to Online Modes Is Changing Education
We live in a time when online learning is students’ main component of education. A huge amount of information regarding students ‘ online activity, as well as their grades, is collected and stored. This huge amount of data allows AI algorithms to offer experiences that were not available prior. Below are a few major ways that AI improves the learner’s experiences of learners:
Individualization is likely the main use case of AI in education. Students accessing educational content via online platforms produce huge data trails. AI models then use the data to generate suggestions, suggest learning pathways, and provide assistance.
Data retrieval for students
They are faced with abundant information sources in this digital age. It’s difficult to pinpoint the documents that will aid them in answering their questions, and keeping students engaged in the process is difficult. Natural processing of language, a sub-field of AI, helps organizations integrate chatbots into their platforms for learning. Chatbots are efficient in locating data that corresponds to students’ inquiries. This method of obtaining information through asking questions rather than searching for answers has been tested to improve the learning experience.
Enhance engagement Increase engagement
While students are on one side of the spectrum, instructors and course creators are on the other. It is also crucial for teachers to make data-driven decisions. AI and big-data systems can collect and analyze usage patterns and reveal the hidden behaviors of their most productive and enthusiastic students. The information can be utilized to enhance students’ learning experiences.
What makes AI crucial for personalizing the learning process in higher education?
The main difference between great and good teachers is that the best teachers understand that every student is unique and can modify learning strategies for students based on their abilities and preferences. Recent advances in AI allow this to be done on a massive scale. Teachers can likely personalize learning to a certain extent; however, what happens if your class comprises many thousands of pupils? Manual methods for personalized learning for open courseware, also known as MOOCs (Massive Open Online Courses), are not possible, but AI will help you develop customized learning strategies. Data is generated each time a student watches the video, participates in class, does not participate, submits an assignment, or submits a project. This information can be used to predict the paths that will allow students to succeed best.
What is the best way to make AI help improve the quality of higher education, offline and online?
Two methods can be used to make use of AI. The first is by creating AI-driven solutions and employing AI to gain insights. Making an AI-driven product involves gathering the data, preparing the data with the Al algorithm, and then developing predictions based on the lessons learned from the model. The inputs for the model are fed to the model via the online software platform. The information then goes to the model trained. In an offline setting, a statistician or data scientist will employ the trained model to gain insights and create scenarios that simulate what-if. Online education has grown from a concept that was initially developed to a real-world application in the last decade. Online education has allowed organizations to tailor and provide customized content to students. This is accomplished by monitoring the user’s interaction patterns, such as test scores and user engagement. AI is also beneficial for offline platforms in which data-driven insights play an important role.
For example, AI can help predict the student’s performance about their participation, attendance, and scores. The early detection of future performance could help colleges and universities create programs to assist students in succeeding.
What are the skills needed to begin a career in Data Science?
The modern data scientist is expected to possess a broad range of abilities. However, that doesn’t mean you have to be an expert in every one of these skills. There are a variety of core skills and other skills to be able to.
Essential skills
Data analysis and analysis, programming, statistics, Machine Learning, Data visualization, data inference, and business expertise. Additional/nice to have Model deployment Big Data Frameworks, advanced machine learning techniques such as reinforcement and deep learning.
Soft abilities
Storytelling, communication outcomes, thinking critically and solving problems. Any suggestions for those who would like to begin with a job in Data Science.
Data scientists who are aspiring should improve their critical thinking abilities. Before you design models, consider the data you have that will explain the variation, analyze those gaps, assess the sources of bias within the dataset, and pay careful focus on what success means to your company and your customers. Understand the basic components of machine-learning algorithms. Most data scientists compare Statistics with the R programming language and Data Science with the Python programming language. Most of the time, Data science is a tool or agnostic programming language. Machine learning models comprise a myriad of mathematical formulas that attempt to reduce error. However, the majority of them contain certain assumptions in the models. It is important to develop a clear opinion about the inner workings of these algorithms before jumping into programming.
What are the jobs available in AI, Data Science?
The areas that comprise Data Science and Artificial Intelligence have seen a significant increase over the past few years. The terms used to describe these fields aren’t always clear. In general, AI is closely integrated with various functions of the enterprise:
AI engineers/Data Scientists are responsible for identifying the required data to build the model, cleaning the data, looking for areas of data that are missing, constructing AI or machine-learning models and then evaluating the outcomes. The primary goal of data scientists is to create reliable statistical data that can be used to make generalizations.
Data Engineers
Data engineers create big data pipelines and information pipelines. The data required for AI models are often stored in various databases. Data engineers create channels that aggregate the data. They also play a vital part in ensuring that the data is model-ready and is easily consumed by models. Data engineers also fulfill crucial tasks of confirming the quality of data.
Analytics
Analysts analyze data with the help of business intelligence and SQL tools. Data analysts’ work is extremely closely aligned with the result of a data scientist. The major difference is that data analysts gain insights through descriptive analytics rather than machine learning. Some roles like business analysts, database administrators, and MLOps developers are also closely related to data science’s function.