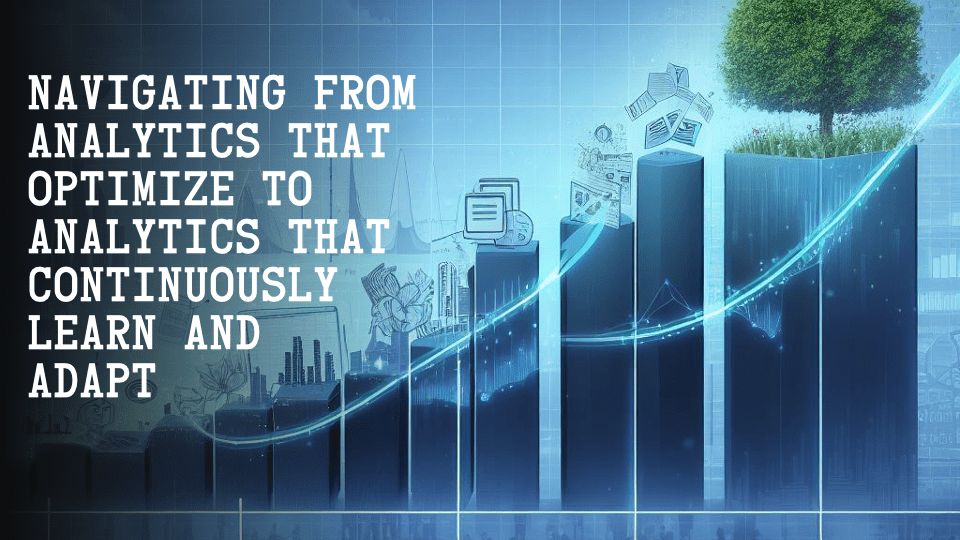
We are living in a time of unprecedented change and innovation. Generative AI (GenAI) has created new horizons for us to explore the possibilities of AI in creating novel and diverse content. But the real revolution lies in the next step of the journey – autonomous analytics, an emerging category of analytics that can learn, adapt, and act with minimal human intervention as they interact with their environment. Autonomous analytics can bring transformative benefits to every aspect of society, including enhancing the availability and quality of healthcare, tackling environmental challenges, addressing transportation safety and bottlenecks, accelerating manufacturing excellence, expanding entertainment innovation, fostering social and economic equity, and much more (Figure 1).
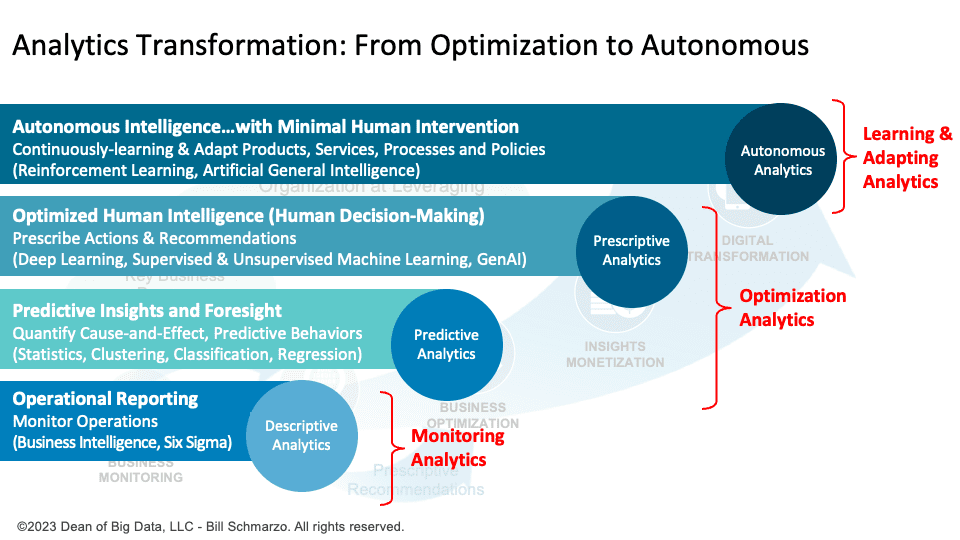
Figure 1: Analytics Transformation: From Optimizing To Autonomous Analytics
What are Autonomous Analytics?
First, some definitions:
- Traditional analytics is the process of collecting, processing, analyzing, and visualizing data to generate insights and recommendations for decision-making. It requires human intervention to define the analysis’s goals and methods and supervise outcomes. Traditional analytics relies on rules and models that must be manually updated and adjusted as market, economic, business, and social conditions change. Examples of traditional analytics applications include Business Intelligence, regression analysis, association rules, clustering, segmentation, data mining, and machine learning.
- Generative AI (GenAI) is a type of AI that can create new data or content, such as text, images, music, code, etc. GenAI uses generative models that learn the probability distribution of the data they are trained on and can sample new data from that distribution. GenAI can be used for various purposes, such as data augmentation, content creation, and data analysis.
- Autonomous Analytics is a type of AI that can learn and adapt to its environment and make optimal decisions with minimal human intervention. Autonomous Analytics is often based on reinforcement learning (RL) that learns from experience and feedback. Autonomous Analytics can be used for various purposes, such as self-driving cars, robotics, complex games, and dynamic optimization problems.
- Artificial General Intelligence (AGI) is a hypothetical form of AI that can achieve or surpass human intelligence across all domains and tasks without being limited by specific goals or contexts. AGI can learn from any data and experience and can transfer its knowledge and skills to new situations it has not encountered before.
GenAI provided a massive leap forward in analytic capabilities unimagined one year ago. Autonomous analytics has the potential for an even more significant leap forward in the quest for Artificial General Intelligence (AGI) or “Super Intelligence.” Autonomous analytics provide the following benefits:
- Autonomous analytics can automatically discover the optimal methods, techniques, and pathways to achieve desired outcomes based on the data and feedback.
- Autonomous analytics dynamically update and adjust its rules and model weights based on the learning and adaptation process.
- Autonomous analytics can support dynamic, constantly changing, and complex operational situations.
- Autonomous analytics can adjust more quickly to changing operational conditions to deliver more accurate and relevant results and actions.
- Autonomous analytics leverages a real-time feedback process to learn and adapt from the results of every decision it makes.
The key to autonomous analytics is the analytics feedback loop. The analytics feedback loop assesses the results of the analytic outcomes (i.e., comparing predicted versus actual outcomes), identifies and codifies learnings from the outcome’s assessment, and feeds those learnings back to the analytics models to update and adjust the model’s weights automatically. This feedback loop enables the autonomous analytic models to learn from each decision and interaction, update the model parameters and weights based upon those learnings, and adapt to new environmental and operational situations with minimal human intervention (Figure 2).
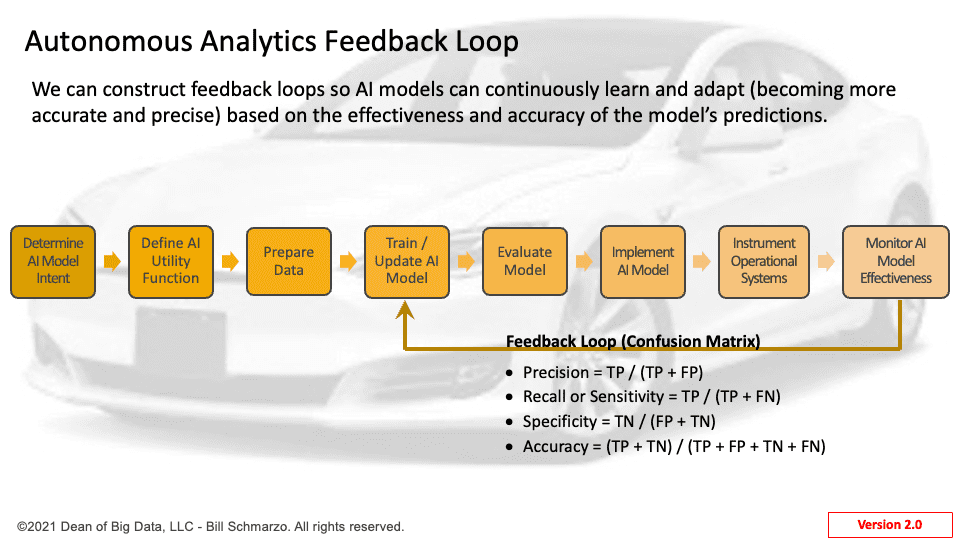
Figure 2: Autonomous Analytics Feedback Loop
Autonomous Analytics can handle complex and dynamic operational situations, provide faster, more accurate, and more relevant outcomes, reduce human effort and error, and create new customer, product, service, and operational value creation opportunities.
What are some Autonomous Analytic Use Cases?
Maybe the best way to envision the potential of autonomous analytics is through a few industry use cases:
- Manufacturing: optimize the manufacturing process by monitoring the production data, detecting anomalies, predicting failures, and adjusting the parameters in real time.
- Healthcare: improve healthcare quality by analyzing medical data, diagnosing conditions, recommending treatments, and providing personalized medical advice and treatments to patients.
- Retail: enhance the customer experience by generating personalized content, such as emails, ads, and social media posts, based on customer profiles and preferences. It can also optimize the marketing strategy and improve customer retention by analyzing customer feedback and behavior.
- Transportation: enable autonomous vehicles by processing the sensor data, recognizing the objects, planning the routes, and controlling the actions. It can also reduce traffic congestion and accidents by coordinating the vehicles and optimizing the traffic flow.
- Agriculture: optimize crop yield and quality by monitoring the soil, weather, and plant data, detecting pests and diseases, predicting harvest time, and adjusting irrigation and fertilization.
- Energy: improve energy efficiency and reliability by analyzing the power grid data, forecasting demand and supply, detecting faults and outages, and controlling generation and distribution.
- Tourism: enhance the travel experience by generating personalized recommendations, such as destinations, activities, and hotels, based on the traveler’s data and preferences. It can also optimize the travel itinerary and budget by analyzing the travel data and feedback.
- Security: prevent and detect cyberattacks by processing network data, identifying anomalies and threats, predicting vulnerabilities, and taking countermeasures.
- Smart Cities: optimize urban services and infrastructure by monitoring the data from sensors, cameras, and IoT devices, detecting anomalies and emergencies, predicting traffic and demand, and adjusting the parameters and policies in real-time.
- Smart Hospitals: improve the quality and efficiency of healthcare by analyzing the data from medical records, devices, and wearables, diagnosing the conditions, recommending treatments, and providing personalized feedback and care to the patients.
- Smart Manufacturing: enhance the productivity and quality of manufacturing by analyzing the data from machines, processes, and products, detecting faults and failures, predicting maintenance and performance, and controlling operations and actions.
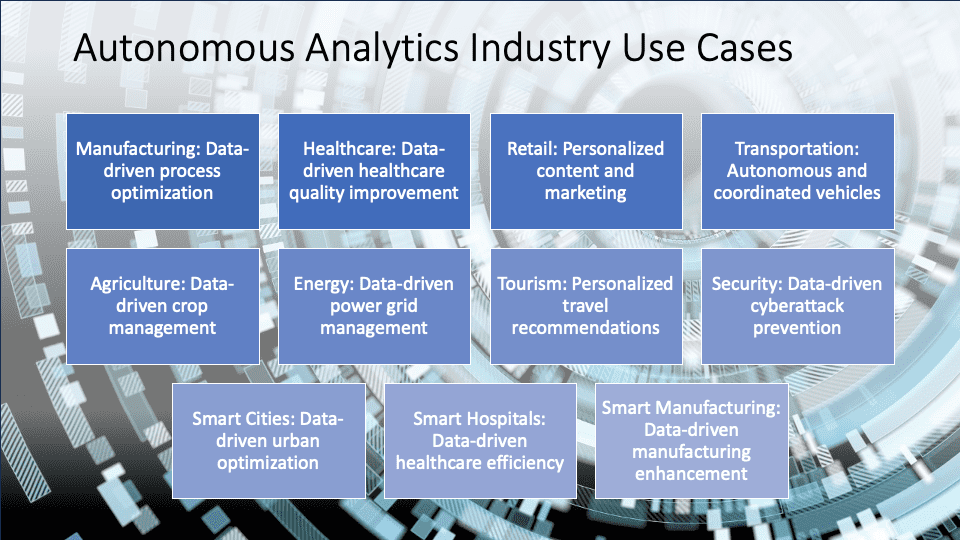
Yeah, it’s pretty cool what could be coming our way!
Summary: Autonomous Analytics
Generative AI has opened our eyes to the fantastic possibilities of AI in creating novel and diverse content. And the next big breakthrough lies in autonomous analytics, an emerging category of analytics that can learn, adapt, and act with minimal human intervention as it interacts with its environment.
Autonomous analytics is the ultimate goal of digital transformation, where organizations create a continuously learning and adapting culture, both AI-driven and human-empowered, that seeks to optimize AI-human interactions and leverage customer, product, and operational insights to create new value and opportunities. Traditional analytics, which focuses on reporting and optimizing specific use cases, are not enough to cope with the dynamic and complex challenges of the 21st century. Instead, we are witnessing the emergence of a new family of analytics that is more focused on learning and adapting than just optimizing.
Autonomous analytics can impact all aspects of our lives – healthcare, education, housing, employment, entertainment, safety, equity, and the environment – if we are rigorous in understanding the problem we are trying to solve, the desired outcomes, and the KPIs and metrics against which we will measure outcomes’ progress and success.
Like any new shiny tool, GenAI and Autonomous Analytics are a means to an end, not an end in themselves. As always, begin with that end in mind.