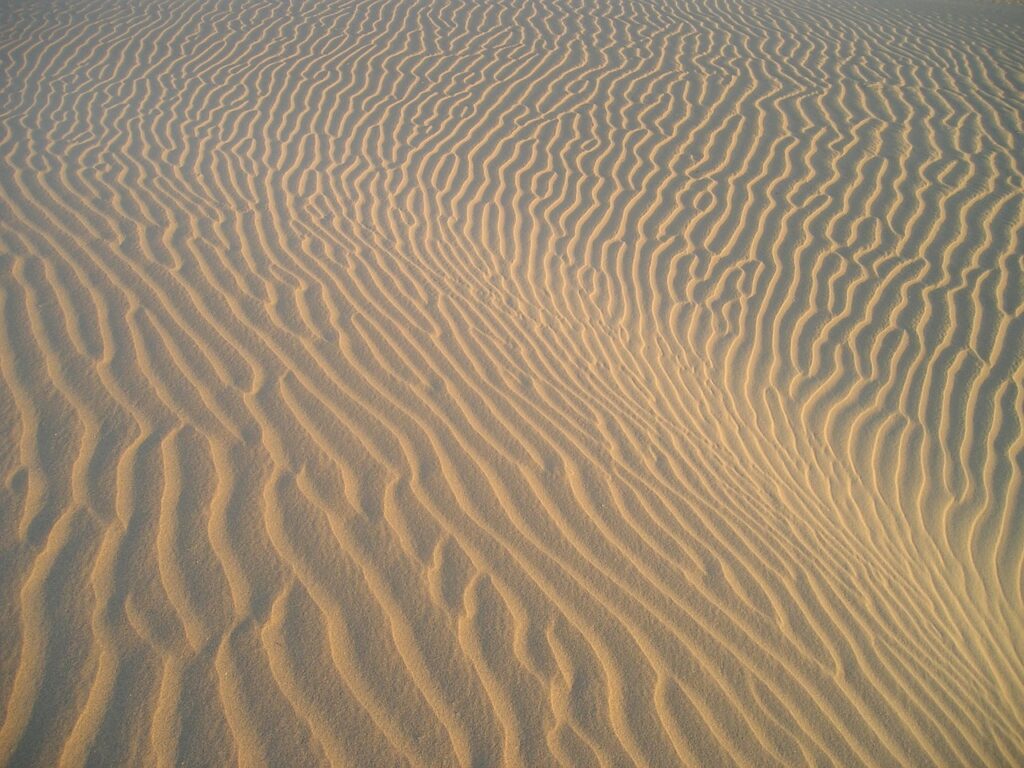
In this two part discussion, we will discuss two related generative AI megatrends
- What are the implications of GPT-4 in terms of model drift
- What is the impact of this limitation on the update of open source LLMs
Backgroumd
A recent paper How Is ChatGPT’s Behavior Changing over Time? from Stanford University and UC Berkeley claims that the performance of GPT-4 has drifted over time. To make this claim, specific tasks were evaluated (ex: accuracy of maths) and the results indicated that for these tasks, the performance degraded from March to June. Data drift in ML models is not new. LLMs are particularly susceptible to drift and other issues related to data due to the manner in which they work i.e. after processing a user query, they leverage the training data to understand the context. They then simply attempt to predict the text output based on this content. This means, they can always get a response even if its incorrect ie there is no direct validation of the response (say in classification or in regression)
Analysis
- In a technical sense, Drift is different from degradation.
- The model is not uniformly getting worse – but rather is getting worse in specific tasks
- There was a seperate an unrelated report of a drop in traffic for GPT models.
- It is possible that because more people are using the system, they are reporting more problems.
- It is claimed that this drift shows that LLMs are a long way from achieving AGI.
- Many of these users trusted generative AI solutions to the extent that they were willing to seek financial, medical, and relationship advice from a virtual assistant.
But the most serious finding is that: because chatGPT is a black box model, users will not trust it to build systems because they cannot understand and quantify the nature of the drift.
While this is indeed serious, this discussion also misses the point that LLMs may not necessarily be used in customer facing situations i.e. many LLMs will be used to assist humans or within the development stack where their output may not necessarily be directly exposed to humans.
Image source: drifting sands over time https://pixabay.com/photos/india-desert-sand-pattern-sand-355/