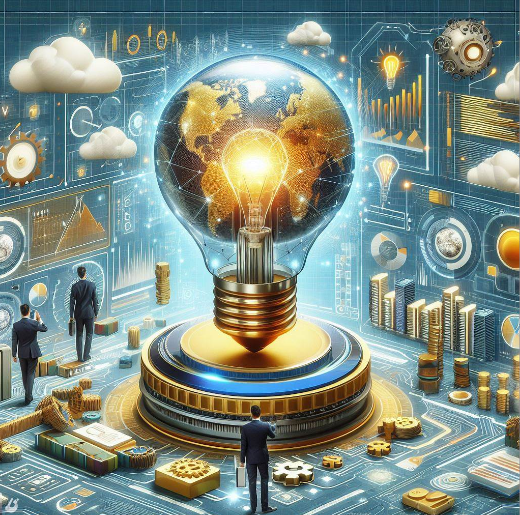
The evolution of data management has kept pace with the rapid increase in data generation, and after beginning with straightforward relational databases and ETL, big data and unstructured data paved the way for the development of automated data pipelines and lakes.
But this data cascade appears to have no stop in sight. Contemporary data surpasses the capabilities of traditional technology due to its complexity, high degree of unstructured nature, and diversity of sources. Thankfully, AI is here to help us with our data management problems.
The fusion of AI & data management
2023 saw the release of generative AI, which accelerated the use of AI. A third of participants in the most recent McKinsey poll indicated that at least one business function is using generative AI. 40% of the corporations reported using AI, and their businesses plan to increase their AI spending.
It’s critical to recognize that data requirements have changed in tandem with the advent of AI in data management. Data sharing is quickly spreading throughout society. Companies want to decentralize their data and provide it as a product to their clients. Furthermore, the market is looking for solutions that allow for enhanced and automated data integration due to the growing need for data fabric.
What then occurs in the background? How is artificial intelligence implemented? Artificial intelligence (AI) technology, such as machine learning algorithms, can speed up repetitive operations like clustering, data purification, anomaly detection, and classification. In addition, text analysis, sentiment analysis, picture analysis, and so forth are made simpler by deep learning and natural language processing.
How is AI impacting data management?
To show how AI affects data management, let’s dissect each stage.
Data extraction
Data extraction is the first phase in any cycle of data management. With unstructured data sources like Text, PDFs, pictures, and more, it has grown more difficult for conventional tools to handle. At first, you could automatically extract data from documents that adhered to the same pattern using template-based techniques.
However, AI has done away with the requirement for templates to be consistent. Natural language processing uses AI-powered data extraction systems to comprehend the fields that companies need to extract. For instance, a company only needs to designate the domains, and the application will pull client data from purchase orders or invoices, independent of the format.
Data mapping
After extraction, the data is mapped from the source to the intended location. It used to be a manual procedure where IT specialists would write code. Data experts may now visualize and perform data mapping with a simple drag and drop thanks to the rapid development of code-free data mapping tools. These days, AI has changed data mapping.
Artificial Intelligence has made it possible to automatically discover data sources, properties, and linkages. Machine learning algorithms save time and effort by analyzing recent data to find connections and trends. Furthermore, AI streamlines the process of mapping schemas since computers employ semantic analysis and pattern recognition to find commonalities between dissimilar schemas.
Data quality
Even while companies are becoming specialists in producing large amounts of data, they still have problems with data quality. IBM estimates that the annual cost of inadequate data quality in the US is $3.1 trillion, demonstrating how little progress is achieved despite the advancement of data management tools. AI, though, may turn out to be unique.
AI systems can quickly identify and fix mistakes, inconsistencies, and abnormalities in datasets. The ability of AI systems to manage missing data is one of its unique features. Without sacrificing precision, AI algorithms can identify missing values in the data and replace them with approximations.
Data analysis
Data analysis, the final stage of any data management process, is where AI may potentially make the biggest impact. Following GPT’s release, NLP integrations in data analytics have become more commonplace. Textual information from documents, social media, and consumer reviews is analyzed using NLP algorithms. Using clustering techniques, AI is also able to combine related data.
In data analysis, two essential methods are regression analysis and decision trees. Even with datasets with several dimensions, sophisticated decision trees can be effortlessly generated by AI-driven machine learning algorithms.
Some challenges while adopting AI in data management
For any new platform to perform to its fullest the ideal requirements must be met. Before organizations can successfully become data-driven, they must overcome a few major adoption and implementation difficulties.
- Vintage architecture
Organizations frequently struggle with the silos that make up their infrastructure. They often aren’t integrated, which prevents them from cooperating well. The data contained in these silos becomes challenging to access. As a result, fewer individuals have access to the data than would be the case if a company invested in more recent technology. Making the switch to the cloud is a crucial step toward improved infrastructure.
- Shortage of talent
Numerous publications demonstrate the persistent lack of skilled personnel in the data science domain. As data engineering processes become more automated, there’s a growing demand for broad skills in data science, machine learning, and artificial intelligence. The younger generation of professionals has to stay up to date with the wide range of abilities needed for data science.
- Changing the mindset
The management team should focus on reorienting the organization’s mentality to embrace new data, analytics, and automation processes. Employees must be comforted that their jobs are not in danger since AI systems free up skilled workers to perform difficult tasks that have the potential to ignite market disruptors. Of course, upgrading skill sets and specific knowledge are necessary. A shift in perspective needs to start with the C-suite and trickle down to every person in a company.
Final Thoughts
In conclusion, businesses face both opportunities and challenges as a result of AI’s impact on enterprise data management. Artificial intelligence (AI) can improve data quality, automate analysis, and expedite data processing, enabling effective data-driven decision-making.
However, integrating AI into data management also necessitates a considerable investment in personnel and technology, as well as adjustments to current workflows and business processes.
For enterprises to fully reap the benefits of artificial intelligence (AI) in enterprise data management, these issues must be carefully considered, and comprehensive plans for successful deployment must be developed.