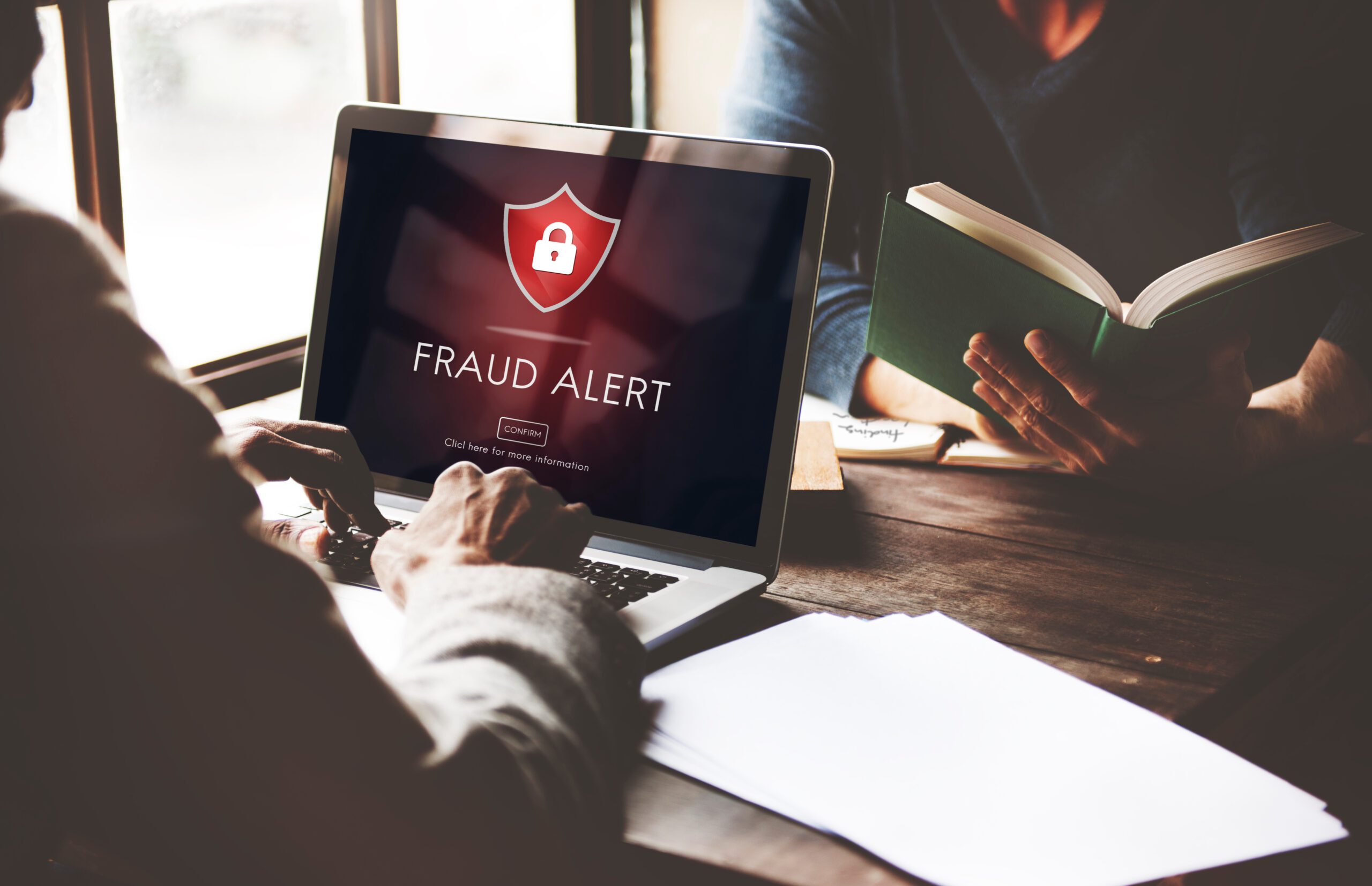
In an increasingly interconnected world where digital transactions have become the norm the battle against fraud has taken on new dimensions. The challenge lies not only in identifying familiar fraud patterns but also in unearthing the intricate web of evolving deceptions that threaten industries such as finance, e-commerce, and insurance. As fraudsters continually adapt their tactics, the need for advanced, dynamic solutions is more critical than ever.
Enter the realm of machine learning—a transformative force that empowers us to combat fraud with unprecedented efficiency and accuracy. In this blog post, we delve into the captivating world of “Fraud Detection using Machine Learning.” We unravel the complexities of detecting deceitful activities in various domains and unveil the cutting-edge techniques that have reshaped the landscape of security.
In the pages that follow, we’ll explore the fundamental principles that underlie the intersection of machine learning and fraud detection. From dissecting the intricate layers of fraud patterns to discussing the nuances of data preparation, feature engineering, and model evaluation, we embark on a journey that unearths the hidden insights locked within the vast troves of data. With every keystroke, we demystify the algorithms that form the bedrock of our fraud-fighting arsenal, enabling us to distinguish between legitimate transactions and fraudulent maneuvers.
As the curtain lifts on this narrative, we’ll not only explore the techniques that have proven effective in historical contexts but also peer into the future. We’ll contemplate how real-time fraud detection is revolutionizing the security landscape, paving the way for proactive intervention. Moreover, we address the ethical considerations that arise in the pursuit of identifying deceit, striving to ensure that our AI-powered solutions remain fair, unbiased, and just.
In a world where deception grows increasingly sophisticated, our pursuit of truth and security is fortified by the capabilities of machine learning. Together, let’s navigate the currents of data, algorithms, and innovation, as we unravel the intricate threads of fraud and emerge with a renewed sense of vigilance and confidence.
Understanding fraud patterns
In the intricate dance between deceit and vigilance, the first step towards effective fraud detection lies in comprehending the dynamic landscape of fraudulent activities. Fraud, a chameleon-like phenomenon, assumes various forms across industries, making it imperative for us to recognize the multifaceted patterns it takes.
The world of financial transactions, for instance, experiences a range of fraudulent behaviors, from seemingly innocuous anomalies to elaborate orchestrated schemes. Payments originating from unfamiliar geolocations or transactions deviating significantly from user spending patterns are just a glimpse of the subterfuge that occurs. Similarly, e-commerce platforms contend with fake accounts, account takeovers, and the ever-elusive “friendly fraud.”
But fraud detection transcends these sectors, extending its influence into healthcare, insurance, and beyond. The clever manipulation of identities in insurance claims or the manipulation of patient records for personal gain illustrates the gravity of this challenge.
The evolution of fraud patterns adds a layer of complexity. Fraudsters adapt to changing circumstances, devising new tactics to stay one step ahead of traditional detection methods. This constant evolution underscores the urgency of adopting advanced techniques that can detect novel fraud patterns and anomalies. This is where the power of machine learning comes into play, enabling us to harness the capabilities of algorithms to dynamically adjust and learn from emerging patterns, staying agile in the face of an ever-shifting landscape of deception.
As we venture deeper into the realm of machine learning for fraud detection, we’re poised to unmask these deceptive patterns and reveal the strategies that keep industries secure. The next stage of our exploration takes us into the heart of data preparation, where we lay the groundwork for the algorithms that form our line of defense against fraudulent activities.
Data preparation
In the quest for uncovering hidden insights within the labyrinthine realm of fraud detection, meticulous data preparation forms the bedrock of our analytical journey. Just as a masterful painter prepares their canvas with care, so too must we lay the foundation for our machine learning models with precision and thoughtfulness.
As we embark on this stage, the sanctity of data quality takes center stage. A symphony of features, labels, and historical data orchestrates our understanding of fraudulent activities. Ensuring that this data is accurate, complete, and devoid of errors is paramount. By rectifying inconsistencies, handling missing values, and refining our dataset, we mitigate potential pitfalls that could mislead our models.
Equally important is the concept of feature engineering—a process where raw data is transformed into insightful variables that illuminate the underlying dynamics of fraud. Characteristics such as transaction amounts, geographical origins, timestamps, and device identifiers take center stage as the building blocks of our analytical arsenal. By sculpting these features thoughtfully, we create a dataset that resonates with the distinct fingerprints of fraud, allowing our models to discern the subtle patterns that elude human observation.
It’s worth noting that this data preparation is not a mere prelude but a meticulous craft that significantly influences the efficacy of our entire endeavor. The integrity of our analysis hinges on the diligence applied at this stage, as the conclusions drawn and the actions taken will echo the meticulous groundwork laid here.
As we proceed, the spotlight shines on the art of feature engineering. We explore how these variables are tailored to the specific challenges of fraud detection, enabling our models to decipher signals from the noise. Join us as we venture deeper into the heart of machine learning, where algorithms and data converge to form a fortress against deceit.
The subsequent segment leads us through the selection of the most relevant features, a process that paves the way for our models to operate with clarity and precision. Stay with us as we navigate this intricate labyrinth of data preparation, and unveil the canvas upon which the masterpiece of machine learning fraud detection will be painted.
Feature engineering
As we traverse the terrain of fraud detection, our journey leads us to a realm where raw data metamorphoses into the currency of insights: feature engineering. Just as a jeweler carefully crafts precious gems into exquisite ornaments, so must we shape our data into refined features that unlock the hidden truths within.
In this symphony of fraud detection, each feature plays a distinct note, harmonizing to reveal patterns that may otherwise elude human perception. Think of transaction amounts, time of day, geographic origins, and the intricate interplay between these variables as the instruments in our ensemble. It is through these well-crafted features that we capture the essence of fraudulent behavior, tracing its nuanced footprints across the data landscape.
However, crafting features is not a mere exercise in data manipulation; it’s an artful endeavor. We delve into the heart of our domain to understand the subtleties that set fraudulent transactions apart. A sudden spike in transaction amount, an unusually high frequency of transactions from a specific location, or a pattern of activity that deviates from established norms—all these hold the potential to unravel the enigma of fraud.
Through the lens of machine learning, we transform these raw attributes into vectors of information, enabling our models to discern the significance of each piece of data. The result is a transformed dataset that reflects the underlying dynamics of fraudulent activities, laying bare the patterns that are integral to our mission.
The intricate dance of feature engineering requires a blend of domain expertise and analytical finesse. It is a testament to the evolving nature of fraud detection—an evolving choreography where insights emerge from the interplay of data and algorithms. Our narrative continues as we delve into the world of machine learning algorithms, where these meticulously engineered features find their true purpose, igniting the spark of detection in the heart of our models.
As the curtain lifts on this phase of our exploration, we prepare to unravel the nuances of various machine learning algorithms, each poised to contribute its unique strengths to our endeavor. Stay tuned as we venture deeper into the tapestry of fraud detection, where each thread woven represents a step closer to uncovering the truth behind deceitful activities.
Machine Learning algorithms for fraud detection
Having laid the foundation through meticulous data preparation and artful feature engineering, we now stand at the crossroads of innovation—the realm of machine learning algorithms. Just as a conductor wields a baton to orchestrate a symphony, we deploy these algorithms to conduct a harmonious exploration of fraudulent patterns.
Each algorithm, like a distinct musical composition, brings its unique strengths to the stage of fraud detection. Logistic Regression, the classic virtuoso, navigates the complexities of binary classification with finesse, offering insights into the probability of fraudulent behavior. Meanwhile, the Random Forest ensemble performs a melodious dance of decision trees, amplifying the signal and minimizing the noise within our data.
As the crescendo builds, the power of Gradient Boosting steps forward, creating a virtuoso of predictive accuracy. Its iterative learning process refines the models with each iteration, enhancing their performance. Not far behind is the commanding presence of Support Vector Machines (SVM), drawing boundaries with precision to segregate fraudulent activities from genuine ones.
In the wings, the allure of Neural Networks beckons—a deep learning ensemble capable of unraveling intricate nonlinear relationships within the data. While their complexity demands careful tuning, the insights they reveal can be profound, enriching our understanding of the fraudulent landscape.
These algorithms, like instruments in an orchestra, harmonize to create a symphony of fraud detection. Through training on historical data, they learn the cadence of legitimate transactions and the intricacies of deceitful maneuvers. Yet it’s important to remember that these algorithms are not a one size fits all solution. The choice of algorithm depends on the specific nuances of the problem, the characteristics of the dataset, and the desired trade off between precision and computational complexity.
As we embrace the diversity of machine learning algorithms, our understanding deepens, and our detection capabilities strengthen. Our journey into the heart of machine learning continues, leading us to the realm of model evaluation and validation. Here, we scrutinize the performance of our algorithms against real world scenarios, ensuring that our symphony of fraud detection resonates with accuracy and reliability.
Model evaluation and validation
As our symphony of fraud detection approaches its zenith, we turn our attention to the critical act of model evaluation and validation—a harmonious process that ensures our algorithms perform not as soloists, but as a cohesive ensemble, attuned to the nuances of real-world scenarios.
In this auditorium of analytics, a myriad of evaluation metrics take center stage. Precision becomes our measuring stick for the proportion of correctly identified fraudulent cases among the total predicted cases. Recall, a soloist in its own right, gauges the ratio of correctly identified fraudulent cases to the actual total fraudulent cases—a measure crucial to detecting fraud comprehensively.
But the heart of our performance lies in the F1-score, a metric that strikes a balance between precision and recall, illuminating the path towards a well-rounded model. And then there’s the ROC curve and the Area Under the Curve (AUC)—elegant instruments that help us visualize the trade-offs between true positives and false positives, guiding us in optimizing the decision thresholds of our models.
Yet, evaluation extends beyond isolated metrics. Cross-validation takes center stage—a technique that emulates the ebb and flow of real-world conditions, guarding against overfitting and ensuring our model’s generalizability. By partitioning our data into training and validation sets, we simulate the real-time challenges of detecting fraud in an ever-evolving landscape.
In this journey, we recognize the significance of stratified sampling, maintaining the delicate balance between the classes of genuine and fraudulent transactions. This technique safeguards our models against biased conclusions that could lead to either excessive false positives or missed fraudulent activities.
Amidst these techniques, the quest for robustness persists. Just as an orchestra rehearses tirelessly to fine-tune its performance, we too must experiment with different algorithms and hyperparameters. This process—akin to tuning an instrument—imbues our models with the resilience to withstand the unpredictabilities of the real world.
Our symphony’s crescendo leads us to confront the ethical considerations embedded in fraud detection. Just as an ensemble aligns its melodies for harmony, we align our algorithms for fairness. Ensuring that our models remain impartial and unbiased is an ongoing pursuit, as we navigate the complexities of decision-making and strive to avoid undue consequences.
Dealing with imbalanced data
In the intricate choreography of fraud detection, the delicate balance between genuine transactions and fraudulent activities often tilts towards the former, resulting in imbalanced data. This asymmetry, akin to an uneven dance, can challenge the efficacy of our algorithms, potentially leading to skewed outcomes.
Just as a conductor maintains equilibrium in an orchestra, we too must address this imbalance to ensure our machine learning models perform harmoniously. When the majority class—genuine transactions—dwarfs the minority class—fraudulent transactions—traditional algorithms can inadvertently prioritize the larger class, relegating the detection of fraudulent activities to the periphery.
One elegant solution to this challenge is oversampling the minority class. By synthetically augmenting the dataset with replicated instances of fraudulent cases, we level the playing field. This technique, like assigning additional instruments to a lesser-heard section of an orchestra, enhances the representation of fraud patterns, enabling our models to discern them with greater accuracy.
Conversely, we can opt for undersampling the majority class. Here, we selectively prune instances from the genuine transactions to align their numbers with the fraud cases. This approach, akin to allowing certain instruments to stand out in an ensemble, ensures that fraudulent activities aren’t overshadowed by the sheer volume of genuine transactions.
A refined dance emerges through techniques such as SMOTE (Synthetic Minority Over-sampling Technique) and ADASYN (Adaptive Synthetic Sampling), which create synthetic instances by intelligently interpolating between genuine cases. This strategic augmentation bridges the gap between classes, fostering a balanced rhythm within our data.
However, just as an orchestra needs both harmony and rhythm to captivate its audience, so too does our analytical approach require a judicious balance. Oversampling can lead to overfitting, while undersampling risks the loss of valuable information. A blend of these techniques, coupled with vigilant validation, ensures that our models resonate with accuracy and robustness.
Real-time fraud detection
As our symphony of fraud detection continues to crescendo, the stage widens to accommodate the demands of real-time scenarios—a dynamic arena where every beat matters. Just as a conductor guides an orchestra through intricate tempo changes, we must orchestrate our machine learning models to perform in harmony with the swift rhythm of ongoing transactions.
In this dynamic landscape, mere post-analysis is inadequate. The tempo of transactions demands proactive intervention, akin to musicians adjusting their melodies in response to the audience’s reactions. Real-time fraud detection calls for the convergence of cutting-edge technology and analytical acumen to decipher fraudulent activities as they unfold.
This technological symphony is conducted through the likes of streaming data platforms such as Apache Kafka and Apache Flink. These platforms enable the continuous flow of data, allowing our models to process, analyze, and respond to transactions in real time. Just as a conductor reacts to the orchestra’s performance in the present moment, these platforms facilitate the instantaneous detection and prevention of fraudulent patterns.
The transition to real-time detection introduces a new layer of complexity. Algorithms must make split-second decisions, requiring a delicate balance between sensitivity and specificity. The interplay between precision and recall takes on heightened significance, as false positives and false negatives can have immediate consequences.
Yet, the dance of real-time detection extends beyond algorithms. Ethical considerations, too, step into the limelight. As we strive to uncover fraudulent activities swiftly, we must remain ever-mindful of user privacy and data protection. Just as a conductor tempers intensity with finesse, we must balance the urgency of detection with the respect for individual rights.
Our symphonic journey persists as we delve into the realm of case studies and examples, where the fusion of theory and practice manifests in tangible solutions. Join us in exploring how real-time fraud detection not only safeguards industries but also transforms the landscape of security, ensuring a vigilant watch over transactions in an ever-evolving world.
Ethical considerations and fairness
As our symphony of fraud detection reaches its pinnacle, we are compelled to pause and reflect on the ethical dimensions that permeate this realm of technology and vigilance. Just as a composer infuses emotion into every note, so too must we infuse our algorithms with the ethics and fairness that guide responsible innovation.
The pursuit of fraud detection should not be a mere chase after deceptive activities, but a conscientious journey that upholds the principles of justice and respect for all individuals. As we deploy algorithms to uncover fraudulent patterns, we must remain steadfast in our commitment to avoid perpetuating biases that could unfairly impact certain groups.
Machine learning algorithms, while remarkable in their capabilities, can inadvertently inherit biases present in the training data. Just as a conductor shapes the orchestra’s interpretation, we must guide our algorithms towards fairness and impartiality. By carefully curating training data and employing techniques like fairness-aware algorithms, we ensure that our models make decisions that transcend pre-existing societal disparities.
The consequences of algorithmic decisions ripple through real lives. A false positive—incorrectly labeling a legitimate transaction as fraudulent—can result in financial distress and reputational harm. A false negative—failing to detect a fraudulent activity—can lead to financial loss and security breaches. Just as a conductor balances harmony and tension, we must balance the weight of these outcomes to minimize their impact.
Transparency is another cornerstone of ethical AI. Just as a composer’s score is open to interpretation, our algorithms should be transparent in their decision-making processes. Through techniques like explainable AI, we empower users and stakeholders to comprehend how decisions are reached, fostering trust and accountability.
In this era of digital transformation, we are not merely orchestrating algorithms; we are shaping the landscape of fairness and justice. Just as a symphony evokes emotions, we must evoke ethical responsibility as we continue our journey into the future of fraud detection. Our narrative evolves as we step into the practical arena of case studies, unveiling how the harmony of ethics and technology transforms theory into reality.
Future trends in fraud detection
As our symphony of exploration nears its conclusion, we turn our gaze towards the horizon of possibilities—an expanse where innovation and technology converge to shape the future of fraud detection. Just as a composer envisions new melodies, so too do we envision the harmonies that will resonate in the realm of security.
The landscape of fraud detection is poised for a transformation fueled by emerging technologies. One notable trend is the evolution of AI explainability, enabling us to peer into the decision-making processes of complex algorithms. This transparency not only fosters understanding but also bolsters accountability, ensuring that our models operate in alignment with ethical considerations.
The marriage of blockchain and data science emerges as a sentinel against fraud, creating an immutable ledger of transactions that transcends tampering. This fusion enhances security, transparency, and traceability, illuminating each step of the transaction journey.
Moreover, the realm of quantum machine learning beckons—an intersection where quantum computing amplifies the capabilities of algorithms. With the potential to process vast amounts of data and tackle complex calculations, quantum machine learning could unveil fraud patterns previously hidden in the noise.
As the digital landscape evolves, so too does the threat landscape. The rise of sophisticated fraud tactics requires an equally sophisticated response. The fusion of machine learning and cybersecurity takes center stage, with algorithms analyzing intricate patterns to detect and mitigate cyber threats before they materialize.
The expansion of privacy-preserving techniques ensures that as we combat fraud, we safeguard individual privacy. Techniques like differential privacy allow us to glean insights from data while minimizing the risk of revealing sensitive information.
While these trends promise transformative shifts, they also bring challenges. The complexity of quantum machine learning, the uncharted terrain of blockchain integration, and the nuances of AI ethics pose questions that will demand exploration and innovation.
Our symphonic journey concludes with the realization that the pursuit of fraud detection is not a static endeavor but a dynamic voyage into uncharted waters. Just as a composer’s legacy extends beyond a single performance, so too will the legacy of our insights and solutions reverberate in the ever-evolving landscape of security.
Conclusion
In the intricate symphony of fraud detection, our journey has traversed the realms of data preparation, feature engineering, machine learning algorithms, model evaluation, ethical considerations, and the future horizons of innovation. We’ve explored how these harmonious elements combine to orchestrate a vigilant watch over the ever-evolving landscape of security.
From the careful preparation of data, where the canvas of insights is painted, to the finesse of feature engineering that crafts the instruments of detection, we’ve laid the groundwork for the powerful ensemble of machine learning algorithms. These algorithms, much like the instruments of an orchestra, create a harmonious blend of precision and recall, each playing its unique role in unraveling fraudulent patterns.
Our symphony of exploration has ventured into the realm of model evaluation, where the orchestra’s performance is critically appraised to ensure its resonance in real-world scenarios. We’ve also embraced the ethical dimensions of this landscape, ensuring that our algorithms play a just and unbiased tune, while fostering transparency through explainable AI.
With the crescendo of our journey, we’ve glimpsed into the future, witnessing the fusion of technologies like blockchain and quantum computing with the art of fraud detection. As the digital landscape evolves, so too does the sophistication of fraudulent tactics, underscoring the need for adaptive strategies.
As we lower the final curtain on this narrative, we invite you to recognize the profound significance of this symphony. Beyond algorithms and data, this is a narrative of resilience, vigilance, and ethical responsibility. Just as a composer’s legacy resonates through generations, the insights gained in this exploration will echo through the evolving landscape of security.
In this era of interconnected transactions, where every exchange is a note in the symphony of commerce, the resonance of machine learning and ethical considerations becomes our guiding melody. As we embrace the cadence of change, let us stride forward with an unwavering commitment to security, innovation, and a harmonious future.
Author Name:- Diana Jane
Company:- https://www.viabletree.com/
Author Bio:- Hi, I’m Diana Jane, a writer at Viabletree. I’m deeply passionate about the power of words and storytelling. Crafting compelling content is my forte, and I take pride in creating engaging narratives that captivate readers. At Viabletree, I work alongside a talented team, where I bring my creativity and attention to detail to every project. I believe in the impact of well-crafted writing to inspire and educate. I’m constantly seeking new challenges and opportunities to expand my skills and make a meaningful contribution to Viabletree’s success.