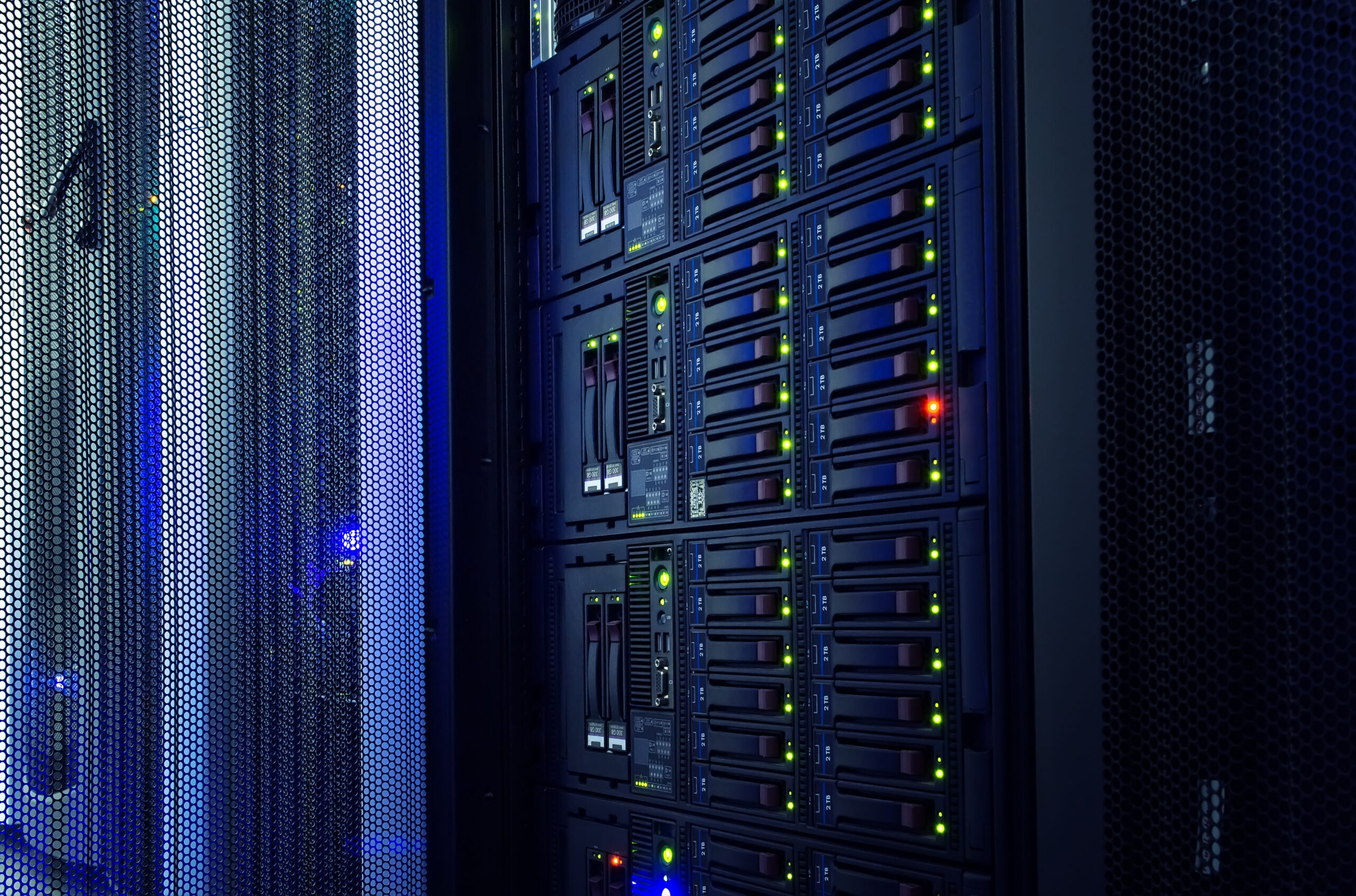
In today’s data-driven world, organizations are constantly seeking ways to unlock the full potential of their data. Data warehousing has emerged as a crucial component in managing and analyzing vast amounts of information. However, with the ever-increasing complexity of data ecosystems, metadata-driven data warehousing, empowered by artificial intelligence (AI), has become the key to unlocking valuable insights and driving actionable business decisions. In this article, we will explore the importance of AI in metadata-driven data warehousing, provide a brief description of the metadata-driven architecture, and outline actionable steps to harness its benefits.
What is a Metadata-powered Data Warehouse? Metadata-driven architecture in data warehousing refers to using metadata to drive various data management and analysis processes. Metadata provides information about data attributes, structures, and relationships, and is crucial in facilitating data integration, governance, and analysis within a data warehousing environment.
Unleashing the power of AI in metadata-driven data warehousing
The rise of Artificial Intelligence (AI) technologies has opened new horizons for further enhancing metadata-driven data warehousing. AI can be a game-changer, augmenting the capabilities of metadata-driven approaches and enabling organizations to extract even more value from their data. Let’s explore how AI unleashes its power in metadata-driven data warehousing:
Automating metadata creation and maintenance
AI can automate the labor-intensive tasks of metadata creation and maintenance, reducing manual effort and improving efficiency. By leveraging AI algorithms, organizations can automatically extract metadata from various sources, such as data schemas, dictionaries, and data profiling results. For example, AI can analyze the structure and content of a database table to infer metadata attributes such as data types, primary keys, and relationships between tables. This automation accelerates the metadata management process and ensures that metadata remains updated.
Improving data quality and accuracy
AI algorithms can enhance data quality through metadata-driven approaches. By analyzing patterns and anomalies in the data, AI can detect data inconsistencies, outliers, and data quality issues that traditional rule-based checks might overlook. For instance, AI can identify data outliers based on statistical analysis and suggest data transformations or cleansing actions. By integrating AI-powered data quality checks into the metadata-driven framework, organizations can proactively address data quality challenges and ensure the accuracy and reliability of the data within the data warehouse.
Enabling advanced analytics and predictive insights
AI empowers organizations to unlock advanced analytics and predictive insights by leveraging metadata-driven data warehousing. AI
algorithms can uncover hidden patterns, correlations, and associations within the data by analyzing metadata attributes such as data types, ranges, and relationships. For example, AI can identify the key factors influencing customer churn by analyzing metadata related to customer demographics, purchase history, and interactions. This knowledge enables organizations to generate predictive models and make data-driven decisions for customer retention strategies.
Enhancing data integration and transformation
AI techniques such as natural language processing (NLP) and machine learning (ML) can streamline data integration and transformation processes in metadata-driven data warehousing. AI-powered NLP algorithms can interpret textual descriptions and user queries, enabling automated mapping between business terms and technical metadata attributes. ML algorithms can analyze historical metadata and usage patterns to suggest data transformation rules and mappings. For example, AI can learn from past transformations and propose the most appropriate transformation logic to convert data from one format to another, saving time and effort in data integration workflows.
A metadata-led approach combined with AI can also improve an enterprise’s data governance capabilities.
Enhancing data governance and compliance with AI
Data governance and compliance are critical aspects of modern data management, and AI technologies offer valuable opportunities to enhance these areas within the context of metadata-driven data warehousing. By leveraging AI capabilities, organizations can ensure regulatory compliance and effectively address data privacy and security requirements. Here’s how AI enhances data governance and compliance:
Automated data classification and tagging
AI can automate classification and data tagging based on its sensitivity, regulatory requirements, and business policies. By analyzing metadata attributes and content patterns, AI algorithms can accurately categorize data into relevant classifications, such as personally identifiable information (PII), financial data, or sensitive intellectual property. For instance, AI can examine the metadata and content of a dataset to identify social security numbers, credit card details, or other sensitive information, enabling automated tagging and appropriate access controls.
Data privacy and security enforcement
AI technologies play a vital role in enforcing data privacy and security measures. With the help of AI algorithms, organizations can proactively identify and mitigate potential privacy risks within their data assets. AI can analyze metadata and content patterns to detect anomalies or unauthorized access attempts, trigger alerts for suspicious activities, and enforce access controls based on data privacy regulations. For example, AI can monitor metadata attributes and user behaviors to identify abnormal data access patterns and detect potential data breaches promptly.
Intelligent data masking and anonymization
AI-powered techniques can facilitate data masking and anonymization processes to comply with privacy regulations while preserving data utility for analytics. AI algorithms can analyze metadata attributes, understand data relationships, and apply intelligent masking techniques to protect sensitive information. For instance, AI can automatically generate synthetic or masked data that retains statistical characteristics while
obfuscating personally identifiable details, allowing organizations to share or use the data in compliance with privacy regulations.
Real-time monitoring and audit trail analysis
AI enables real-time monitoring and analysis of data activities, allowing organizations to track data access, usage, and modifications. By analyzing metadata and monitoring user behaviors, AI algorithms can identify suspicious activities that might indicate data breaches or non-compliance incidents. For example, AI can detect unauthorized attempts to modify or access sensitive data, triggering alerts for immediate investigation and remediation.
Data governance policy enforcement
AI algorithms can automate the enforcement of data governance policies and procedures. By leveraging metadata attributes, AI can evaluate data quality, consistency, and adherence to defined standards. It can analyze metadata to ensure compliance with regulations and policies, such as data retention periods or sharing agreements. For example, AI can assess metadata attributes to validate that data entities are correctly documented, data lineage is maintained, and data usage adheres to defined policies.
Continuous compliance monitoring and reporting
AI technologies can facilitate constant monitoring and reporting of compliance status. By analyzing metadata and tracking changes in regulations or policies, AI algorithms can generate automated compliance reports, highlighting any areas of non-compliance or potential risks. For example, AI can analyze metadata against evolving privacy regulations and develop compliance dashboards that provide an overview of compliance status, identify potential gaps, and recommend corrective actions.
To summarize, AI enhances data governance and compliance efforts within data warehousing. Through automated data classification, privacy enforcement, data masking, real-time monitoring, policy enforcement, and continuous compliance monitoring, AI technologies provide organizations with the tools to ensure regulatory compliance, mitigate risks, and safeguard sensitive data. Organizations can establish robust data governance frameworks by leveraging AI with metadata-driven approaches and maintaining a secure and compliant data environment.
AI as the future of metadata-driven data warehousing
Metadata-driven data warehousing, enhanced by the power of AI, has revolutionized the landscape of data management and business intelligence. The use of metadata as a driving force behind data integration, governance, and analytics has proven to be a game-changer for organizations seeking to leverage their data assets effectively.
Metadata-driven data warehousing opens new possibilities for organizations to unlock the full potential of their data. By harnessing the power of metadata and AI, organizations can drive business intelligence, enhance data governance and compliance, and achieve greater agility and insights.