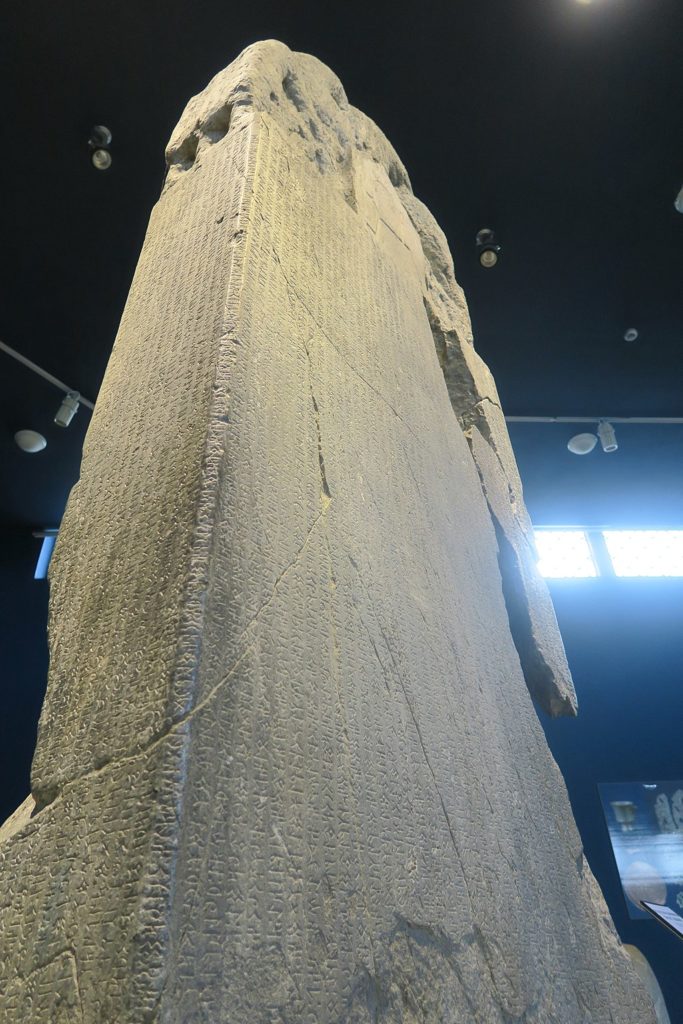
Back before he retired, Naval War College professor and contributor to The Atlantic Tom Nichols published a 2017 book called The Death of Expertise. Those who claim their own facts or knowledge without supporting evidence, he noted, have become more and more prominent in online conversation we’ve been having. And the noisiest and most prone to online pyrotechnics and bomb throwing have been getting the most attention.
As a result, the quieter voices from those who know how to build a balanced consensus–using mutually agreed-upon facts–and have expertise in areas that have a direct and immediate impact on society are being drowned out.
Here’s a recent example of how those with expertise seem to have less clout now than they used to. A team of researchers at the Center for Infectious Disease at Imperial College, London modeled COVID-19 infections and vaccinations using data from 185 countries to get a better sense of the impact of vaccinations on death rates attributed to COVID-19 infections. Their findings? They estimated the vaccines prevented at least 14.4 million deaths globally between December 8, 2020 and December 8, 2021. And like other vaccines, minor side effects from COVID-19 vaccinations are common, but severe reactions are quite rare.
And yet it was necessary for Federal courts to strike down a state law prohibiting COVID-19 vaccination mandates (OSHA being the Federal agency advocating employer vaccine mandates). The law, which even prohibited vaccination disclosure requirements, had been passed by the Montana State Legislature and signed into law by Governor Greg Gianforte in May 2021.
It took until December 2022 for the courts to get to the point in the process where a judge could make a substantive, permanent ruling. Gianforte favored vaccination in the state but opposed a Federal mandate. By July 2021, over half of Republican US House representatives had refused to disclose whether they had been vaccinated against COVID-19 or not.
For at least one of the two major political parties in the US, the expertise denialists got their way. A bizarre situation, considering that George Washington first mandated that Continental soldiers be inoculated from smallpox during the Revolutionary War over 240 years ago.
In 1977, the World Health Organization declared that smallpox had been eradicated worldwide, seeing no further evidence of the disease naturally occurring. 45 years ago, there wasn’t much quibbling about school-age children, active duty military, or healthcare workers needing to get vaccinated. But now there is, with many in power pandering to a minority of the population whose voice now gets amplified by the attention economy.
The provincialism of current data science
Data science should be a broad field of study with all sorts of nooks, crannies and diverse methods from different fields of study playing a role. Instead, it’s narrowly defined, in a way that actually prevents broad, multifaceted progress.
Considering the persistent lack of data maturity in most organizations, we should be thinking and researching in a divergent mode. After all, current data management clearly doesn’t scale, and yet we keep trying small iterations of the same old methods. Entrepreneur and Stanford CS adjunct professor Andrew Ng and others have pointed out numerous times that data is the gating factor in our quest to get beyond narrow AI. But most of the money funding innovation on the data front goes into incremental, rather than transformational, technologies.
That’s not to mention all the different talents and skills that need to be tapped to tackle the challenge of artificial general intelligence (AGI).
Divergent versus convergent data science thinking
The preponderance of today’s data science thinking and research is convergent, even though most lack a basic, sound approach to data collection, transformation, analysis and sharing, one that doesn’t insist on stripping collected data of its essence, or ignoring the need to capture and preserve the context and provenance of the data.
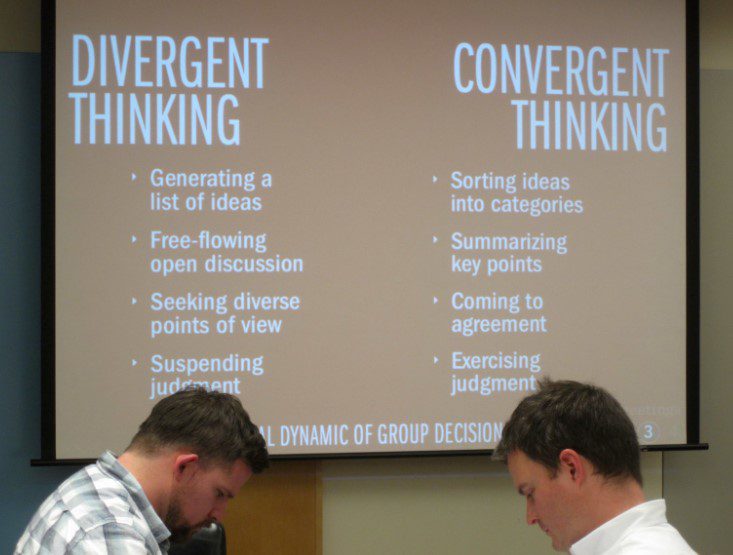
Brace yourself for bigger, better and badder convergent data science thinking in 2023
It’s not like the experts writing articles about data science and AI are exclusively narrow in their thinking. Stanford Fellow and Forbes contributor Lance B. Eliot pondered the current promise and threats of “AI” as we enter the new year in a December 2022 article.
We will see AI that gets bigger in the sense of being more encompassing and able to do things that previously had not been especially viable for AI to do. Bigger also applies to the notion that more people will become aware of AI and there will be increasing worldwide attention to AI.
When talking about better, that’s the watchword for the fact that AI will further amaze us all at how well AI works, seemingly appearing to be somewhat human-like in various ways (leading regrettably to more false claims of AI sentience and other outrageously nonsensical balderdash contentions about today’s AI….) Many pundits and journalists often stop at the mention of AI as becoming bigger and better, thus opting by default or due to AI-illiteracy to not acknowledge the other side of this coin, namely that AI is also going to be badder (shall we say, ostensibly bad to the bone).
We are going to have a lot more AI that is atrocious.
Eliot went on to describe the pros and cons of generative AI, and commented on the buzz he was seeing on social media about ChatGPT, underscoring the amount of hyperbole being posted. Then he mentioned an aspect of current-day AI discourse that particularly resonated:
A type of unfortunate polarization has seemingly invaded AI these days. Here’s what I mean. I just said that generative AI is not sentient. For some people, those are fighting words. They get enraged as though I am denigrating the amazement factor of generative AI. Daring to question the nature of generative AI becomes a kind of triggering point.
Daring to question what the field of data science has settled on is what we should be doing more of, rather than less. I have the same distaste Eliot does for the bullying, denialist aspect of social media debate.
Yes, there are clear breakthroughs, and ChatGPT is a prime example of a breakthrough of sorts. But it’s just a piece of the AI puzzle, and we haven’t even set up the card table properly yet to be able to get all the pieces together in one place.