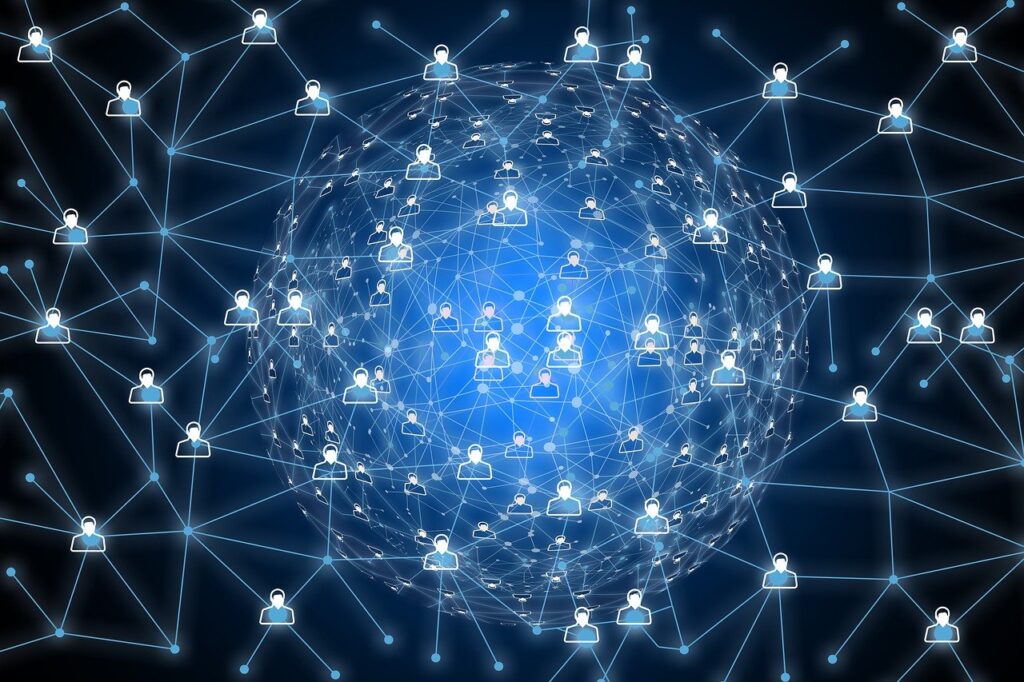
Members of the European Parliament and the Council reached provisional agreement on the Artificial Intelligence Act on December 9th, 2023 after years of debate and discussion. The AI Act is broad in scope and is intended to protect public welfare, digital rights, democracy, and the rule of law from the dangers of AI. The Act in this sense underscores the need to ensure and protect data sovereignty of both individuals and organizations.
On the data sovereignty regulation front, Europe’s approach is comparable to California’s on the vehicle emissions regulation front. Carmakers design to the California emissions requirement, and by doing so make sure they’re compliant elsewhere. “Much like the GDPR [the EU’s General Data Protection Regulation, which went into effect in 2018], the AI Act could become a global standard. Companies elsewhere that want to do business in the world’s second-largest economy will have to comply with the law,” pointed out Melissa Heikkilä in a December 11, 2023 piece in the MIT Technology Review.
What is AI? An updated definition per the OECD
In November 2023, the Organisation for Economic Co-operation and Development’s (OECD’s) Council updated its definition of artificial intelligence. The European Parliament then adopted the OECD’s definition, which is as follows (emphasis mine):
An AI system is a machine-based system that, for explicit or implicit objectives, infers, from the input it receives, how to generate outputs such as predictions, content, recommendations, or decisions that can influence physical or virtual environments. Different AI systems vary in their levels of autonomy and adaptiveness after deployment.
Note the text above in boldface. AI systems infer how to generate outputs from inputs. In other words, AI systems are entirely dependent on the quality of their data input.
We can talk all we want to about trustworthy models, but when it comes to statistical models being trustworthy, inputs rule. High data quality is a prerequisite. When the input is garbage, the output will be garbage also.
Most of the time, data scientists grapple with the input before training their models, so the output they end up with often seems reasonable. But the output despite their efforts can be problematic in ways that aren’t straightforward. How to solve that problem? Make sure the data quality is high to begin with, before it gets to the data scientist. And then make sure the data scientists preserve that quality by preserving context throughout the rest of the process.
Enable explicit machine-understandable context in data to ensure AI Act compliance
The best way to think about ensuring data quality up front is domain by domain. Each business domain needs to produce relevant, contextualized data specific to that domain. Then at a higher level of abstraction, the organization needs to knit that context together to be able to scale data management.
What results is an input model of the business, described as consumable data, that accompanies the rest of the data when fed to machines.
With specific context articulated in the input data, the data becomes explicit enough for machines to associate the data supplied as input with a given context. Explicit relationships stated as facts in domain-specific data are what help to create sufficient context. They’re what distinguishes tennis matches from kitchen matches.
Organizations need to spell things out for machines by feeding them contextualized facts about their businesses. Volumes and volumes of text, systematically accumulated, can deliver bits and pieces of context. But still, a good portion of that context will be missing from the input data. How to solve that problem? Those responsible for each domain’s data can make each context explicit by making the relationships between entities explicit.
Once those relationships are explicit, each organization can connect the contexts for each domain together with a simplified model of the business as a whole, what’s called an upper ontology.
Scaling relationship-rich, quality data with the help of knowledge graphs
Most organizations have been siloing data and trapping relationship information separately in applications because that’s what existing data and software architecture mandates.
Knowledge graphs provide a place to bring the siloed data and the necessary relationship information for context together. These graphs, which can harness the power of automation in various ways, also provide a means of organization-wide access to unified, relationship-rich whole. Instead of each app holding the relationship information for itself, the graph becomes the resource for that information too. That way, instance data and relationship data can evolve together.
Graphs facilitate the creation, storage and reuse of fully articulated, any-to-any relationships. This graph paradigm itself encourages data connections and reuse by contrast with the data siloing and code sprawl of older data management techniques.
Intelligent data in knowledge graphs will help scale AI Act compliance efforts
Intelligent data is data that describes itself so that machines don’t have to guess what it means. That self-describing data in true knowledge graphs provides machines sufficient context so that machines can provide accurately contextualized output. This addition of context is what makes the difference when it comes to AI accuracy. The larger, logically interconnected context, moreover, can become an organic, reusable resource for the entire business.