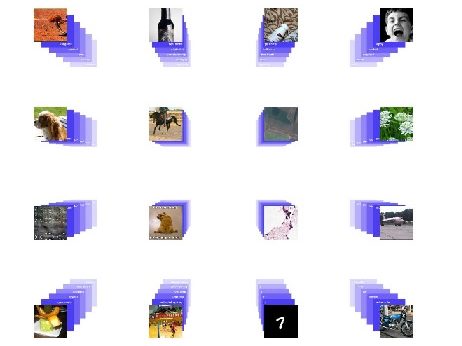
The Stanford HAI (Human cantered artificial intelligence) report is out. I track this report every year and it always has some good insights. The report is a bit more focused on large language models and their impact – but also covers key trends
The main findings are
- Private investment in AI soared while investment concentration intensified:
- U.S. and China dominated cross-country collaborations on AI:
- Language models are more capable than ever, but also more biased:
- The rise of AI ethics everywhere:
- AI becomes more affordable and higher performing:
- Data, data, data:
- More global legislation on AI than ever:
- Robotic arms are becoming cheaper:
In this post, I am going to focus on a specific aspect mentioned in the report – biases in CLIP
CLIP Contrastive Language-Image Pretraining (CLIP) is a powerful technique making a big impact in neural networks. In essence, CLIP learns visual concepts from natural language supervision. CLIP can be applied to any visual classification benchmark by simply providing the names of the visual categories to be recognized, similar to the “zero-shot” capabilities of GPT-2 and GPT-3. General-purpose models such as CLIP (and also ALIGN, FLAVA, Florence, and Wu Dao 2, etc) are trained on joint vision-language datasets compiled from the internet and can be used for a wide range of downstream vision tasks, such as classification.
CLIP learns visual concepts from natural language by training on 400 million image-text pairs scraped from the internet, and it is capable of outperforming the best ImageNet-trained models on a variety of visual classification tasks. However, like other large language models pretrained on internet corpora, CLIP exhibits biases along with gender, race, and age.
Also, unlike computer vision and natural language, there are no benchmarks for measuring multimodal biases in CLIP. This can lead to issues such as Denigration Harm: at the design of categories used in the model (i.e., ground-truth labels) heavily influences the biases manifested by CLIP; Gender Bias i.e. CLIP almost exclusively associates high-status occupation labels like “executive” and “doctor” with men, and disproportionately attaches labels related to physical appearance to women; Propagating Learned Bias Downstream: CLIP has also been shown to learn historical biases and conspiracy theories from its internet-sourced training dataset; Underperformance on Non-English Languages
The innovation created through CLIP and similar algorithms is disruptive.
In my view, the report does a good job of highlighting the challenges for CLIP, these can be overcome.
Report: Stanford HAI (Human cantered artificial intelligence)
Image source: CLIP Contrastive Language-Image Pretraining (CLIP)