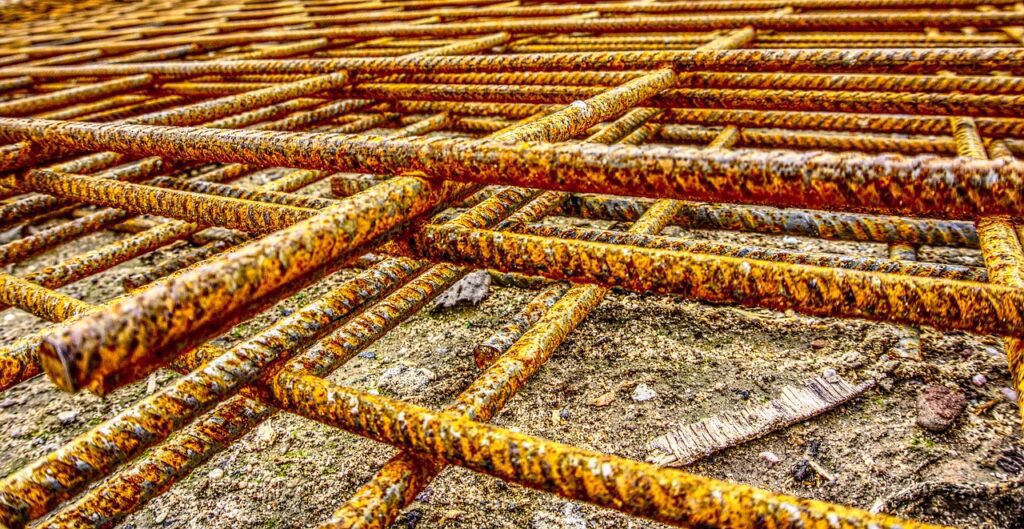
Data science was a vaguely defined discipline to begin with, but it’s shaped up substantially lately. Execs now yearn to take immediate advantage of generative and other clearly useful (if currently problematic) kinds of AI.
That demand suggests an opportunity for influencers and visionaries in organizations to lobby for each organization to build an AI-ready data foundation, one that supports hybrid AI (knowledge graph + statistical machine learning) as well as existing ML modeling efforts. Otherwise, companies won’t be able to scale their AI efforts.
Role articulation needs for the AI-enabled enterprise
Data scientists are busy enough with statistical machine learning models and doing the data prep needed to create useful models. And data engineers are consumed with creating pipelines and tapping and making accessible the resources the data scientists and others need. The specialists staffing these roles are too focused on their own disciplines to worry about the hows and whys of semantic knowledge graphs and architectural transformation overall.
It’s obvious that other roles need to be created or updated to complement the data scientist and data engineering roles. If as Andrew Ng says, better data beats better algorithms, don’t enterprises need to focus on creating the kind of findable, accessible, interoperable and reusable (FAIR) data most suited to large-scale AI efforts? What about the need for knowledge engineers, architects, ontologists, taxonomists and stewards to establish means of ownership and sharing the disparate kinds of FAIR data that’s best managed and scaled via a knowledge graph?
What’s missing from discussion of AI is a suitable data foundation for enterprise-wide AI. Organizations often just don’t think first about how to scale their AI efforts, or even ponder why a foundation is required. Their blind spot seems to reflect a passive attitude about cloud computing and an assumption that clouds are AI-ready to begin with.
The truth is that public software as a service (SaaS) puts the data interests of cloud providers first. To counter that tendency and the fragmentation that comes with thousands of different SaaS subscriptions, enterprises need to stake out much more of their own data territory. They can do that with the help of data-centric (rather than application-centric architecture) and data architects who can guide real AI-scaling transformation.
Multi-purpose architecture and its flexibility benefits
Think for a moment from a building architect’s point of view and the case of a multi-purpose commercial building. Just as today’s new buildings must often begin as multi-purpose, today’s AI must be multi-use. Otherwise, the inefficiency in having to tear down and rebuild the foundation for each use from scratch will become overwhelming.
The building architect’s challenge today is to envision the design of a building that will be flexible in its uses. The trend lately here in the US and perhaps elsewhere as well is to start with the concept of an urban village and assume that most buildings in the village will be suitable for residential, retail and office purposes at a minimum. Some buildings, of course, will continue to be built for one purpose, but those will be the exception, not the rule.
The AI-ready enterprise will need the same kind of foundational flexibility. With a proper, interoperable data foundation–the kind a good knowledge graph can provide–an enterprise can grow its own data, rules and processes from that foundation to suit its AI needs.
Building owners need more and more flexibility to lease out the space they own. These multi-purpose buildings start as shells, with each floor its own tabula rasa for designing what current needs are. As those needs change, parts of each floor can be redesigned to accommodate different kinds of tenants.
For example, here in San Jose where I live, the downtown buildings need to provide more residential than office space, considering that workforces do quite a bit more work from home now. Residential space is at a premium. Google began with one grandiose office + other purposes plan for its downtown San Jose campus before the pandemic. Now, their plans and presumably the demands from the city planning department are changing.
Architects, of course, work with general contractors and their subcontractors–tradespeople with complementary specialties. Those generals and their trades are critical to the effective and efficient execution of any architect’s plan.
Building a multi-purpose data foundation for hybrid, neurosymbolic AI
Knowledge graphs have been around for over a decade now. The technology is mature and extensible. At this point, it’s hard to find a member of the Fortune 50 that hasn’t built a knowledge graph yet. But what is difficult to find is a large enterprise who’s taken its knowledge graph activity and used that graph as a foundation for its larger AI efforts. Most haven’t taken that mental leap yet.
Montefiore Health, a hospital chain in the New York area, is one exception that may prove the rule. Its Patient-Centered Analytics Learning Machine (PALM) is built on a knowledge graph that Franz, provider of the Allegrograph database, helped to engineer and implement.
The Montefiore knowledge graph brings together many critical, but disparate external and internal sources so that machine learning and advanced analytics methods can be run that benefit from the entire connected whole. The PALM as a result can predict and prevent specific occurrences of sepsis and respiratory failure, for example.
Today, GAI efforts are crying out a sounder, more reliable data foundation, one that brings trustworthiness and certainty to users. The term “neurosymbolic AI”, which the AI centers at the University of South Carolina (headed by Amit Sheth) and Kansas State University (headed by Pascal Hitzler) are advancing, captures the complementary nature of the neural nets (statistical deep learning) and symbolic AI (embodied today in semantic knowledge graphs). All we need is to build awareness of the power of these two technologies together so that there’s more wood behind the AI arrow than mere algorithmic or prompt interface magic.