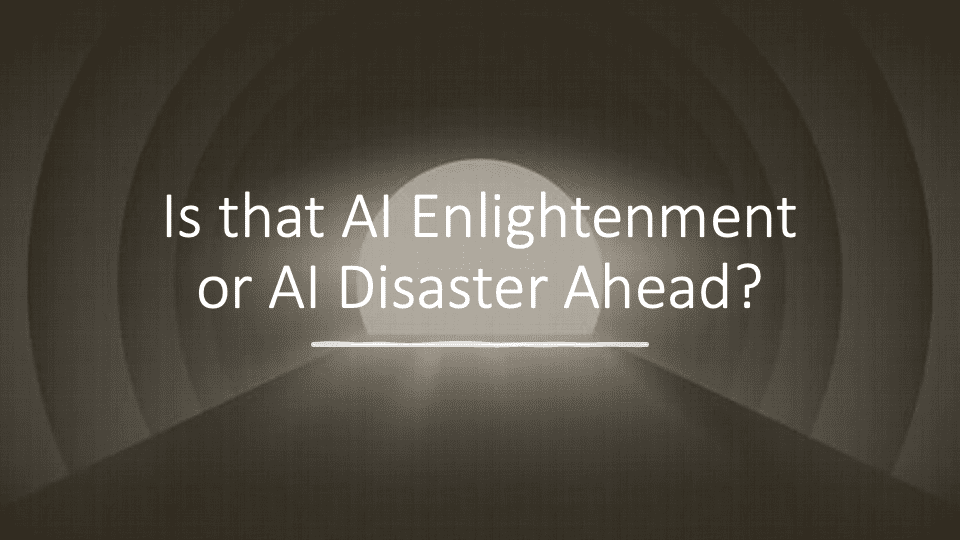
This three-part series outlines the challenges and actions that the Board of Directors for organizations must address as they guide their organization’s responsible and ethical deployment of Artificial Intelligence (AI). Part one will cover mitigating the impacts of AI Confirmation Bias.
Leaders across various business, technology, social, educational, and government institutions are deeply concerned about the potential negative impacts of the untethered deployment of Artificial Intelligence (AI).
In the article “Experts are warning AI could lead to human extinction. Are we taking it seriously enough?” Dan Hendrycks, Executive Director of the Center for AI Safety, states: “There are many ‘important and urgent risks from AI,’ not just the risk of extinction; for example, systemic bias, misinformation, malicious use, cyberattacks, and weaponization.”
There is certainly an immediate need for global collaboration to create policies, regulations, education, and tools that stave off dire actions from nefarious actors and rogue nations who might leverage AI for misinformation, malicious use, cyberattacks, and weaponization.
But there are other equally dangerous risks from the careless application of AI, including:
- Confirmation Bias from poorly-constructed AI models that deliver irrelevant, biased, unethical outcomes and impact longer-term operational viability.
- Unintended Consequences from decision-makers making decisions without consideration of the second and third (and fourth and fifth…) order ramifications of what “might” go wrong.
This is where an organization’s Board of Directors must step up. The Boards across organizations are facing a challenging dilemma caused by the emergence of Artificial Intelligence (AI).
On the one hand, AI can bring compelling benefits to organizations by helping them more quickly exploit changes in their business and operating environments. The thoughtful deployment of AI can improve efficiencies in key business processes, policies, and use cases and reduce compliance, regulatory, and market risks. AI can help organizations uncover new sources of customer, product, service, and operational value while creating a differentiated, compelling customer experience.
On the other hand, AI can have adverse effects on an organization’s long-term viability and unintentionally expose them to compliance and regulatory risks. For instance, AI models that exhibit confirmation bias hinder an organization from attracting new customers or offering new products/services. Moreover, poorly designed AI models can impede necessary operational changes required to adapt to market, societal, regulatory, and environmental changes.
Let’s start this two-part blog series by covering what the Board of Directors needs to understand about Confirmation Bias.
Addressing AI Confirmation Bias
Confirmation bias is a cognitive bias that occurs when people give more weight to evidence supporting their existing beliefs while downplaying or ignoring evidence contradicting those beliefs.
AI model confirmation bias can occur when the data used to train the model is unrepresentative of the population the model is intended to predict. AI model confirmation bias can lead to inaccurate or unfair decisions and is of significant concern in fields such as employment, education, housing, healthcare, finance, and criminal justice, where the consequences of incorrect predictions can be destructive.
Creating AI models that overcome AI confirmation bias takes upfront work…and creativity. That work starts by 1) understanding the costs associated with the AI model’s False Positives and False Negatives and 2) building a feedback loop where the AI model is continuously learning and adjusting from the False Positives and False Negatives. For example, in a job application use case (Figure 1):
- A Type I Error, or False Positive, is hiring someone the AI model believes will be successful, but ultimately, they fail. The False Positive situations are easier to track because most HR systems track the performance of every employee, and one can identify when an employee is not meeting performance expectations. The “bad hire” data can be fed back into the AI training process so that the AI model can learn how to avoid future “bad hires.” Easy.
- A Type II Error, or False Negative, is not hiring someone the model believes will not be successful, but ultimately, that person goes on to be successful elsewhere. These False Negatives are hard to track and require creativity to capture False Negatives data. But tracking these False Negatives is necessary if we want to leverage them to improve the AI model’s effectiveness.
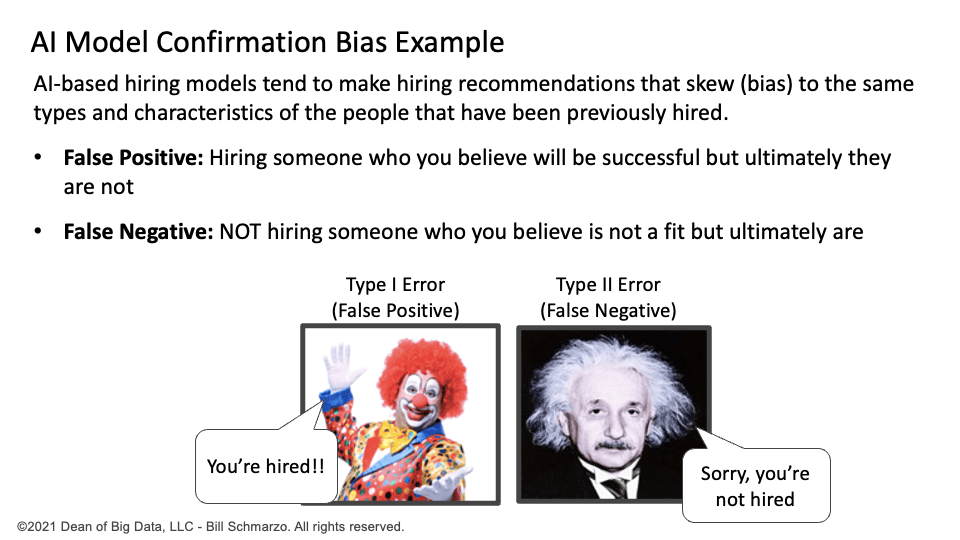
Figure 1: AI Model Confirmation Bias
Another danger is that AI confirmation bias can feed upon itself, creating an echo chamber targeting the same customers and recommendations. AI Confirmation Bias can lead to a decay in your Total Addressable Market (TAM) because your AI models focus on the same customers and recommendations, missing out on opportunities to reach new customers outside the scope of your AI model’s knowledge (Figure 2).
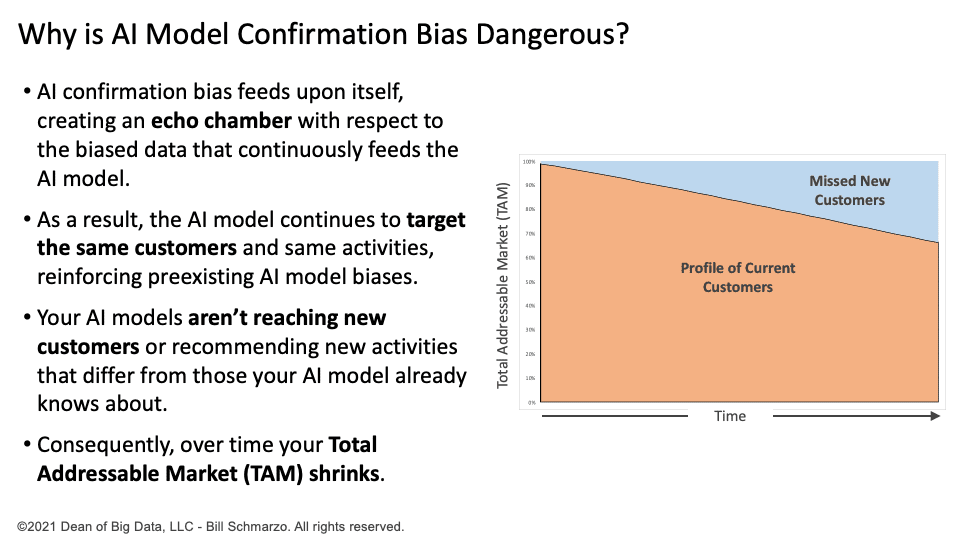
Figure 2: AI Confirmation Bias Can Lead to Shrinking TAM
The good news is that this problem is solvable. We can construct AI models that can continuously learn and adapt based on the effectiveness of the model’s predictions. We can build a feedback loop to measure and provide feedback on the effectiveness of the model’s predictions so that the AI model can get more accurate with each prediction (Figure 3).
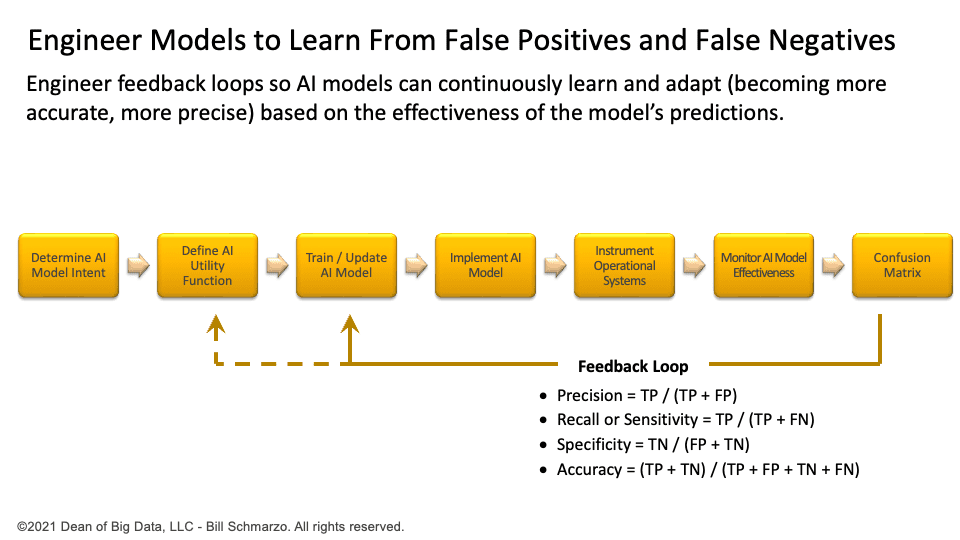
Figure 3: Constructing AI Model Feedback Loop
The Danger of Proxy Measures
Proxy Measures are indirect or substitute variables used to estimate or approximate a target variable of interest.
One deceptive way confirmation bias can sneak into our AI models is through proxy measures that approximate the variable we are trying to omit from the models. Proxy measures become a particular issue when dealing with protected classes[1].
For example, let’s say we are trying to remove the protected class of gender from AI model consideration. Unfortunately, there are many proxy measures in the data that can be indicators of gender (Figure 4).
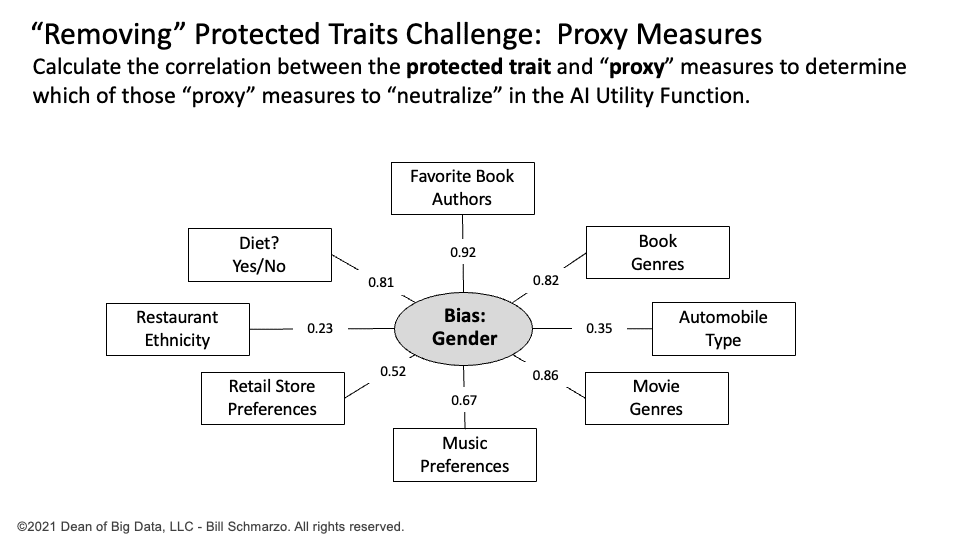
Figure 4: Identifying and Quantifying Proxy Measures
For example, one could easily detect the gender of my wife based on the types of movies watched (Romantic Comedies, Art Films), whether on a diet (always), retail outlet preferences (RealReal, J. Peterman), and favorite book authors (Anne Lamott).
To address the proxy measure problem, we can use knowledge graphs to identify potential “proxy” measures that correlate to the protected trait (Gender) we want to remove from the AI model training and inference data sets. We can calculate the correlation strength between the protected trait and any “proxy” measures to the protected trait to determine which “proxy” measures to remove from the AI Utility Function.
Summary: Board of Directors AI Confirmation Bias Challenge
In part one of this two-part series, we discussed what the Board of Directors needs to know to mitigate the impacts of AI Confirmation Bias.
In part two, we’ll discuss unintended consequences and conclude with a checklist of items that the Board of Directors needs to consider when advising senior management on mitigating the impacts of AI confirmation bias and the unintended consequences of management decisions.
[1] Protected classes include race, color, religion, sex (including pregnancy, sexual orientation, or gender identity), national origin, age (40 or older), disability, and genetic information