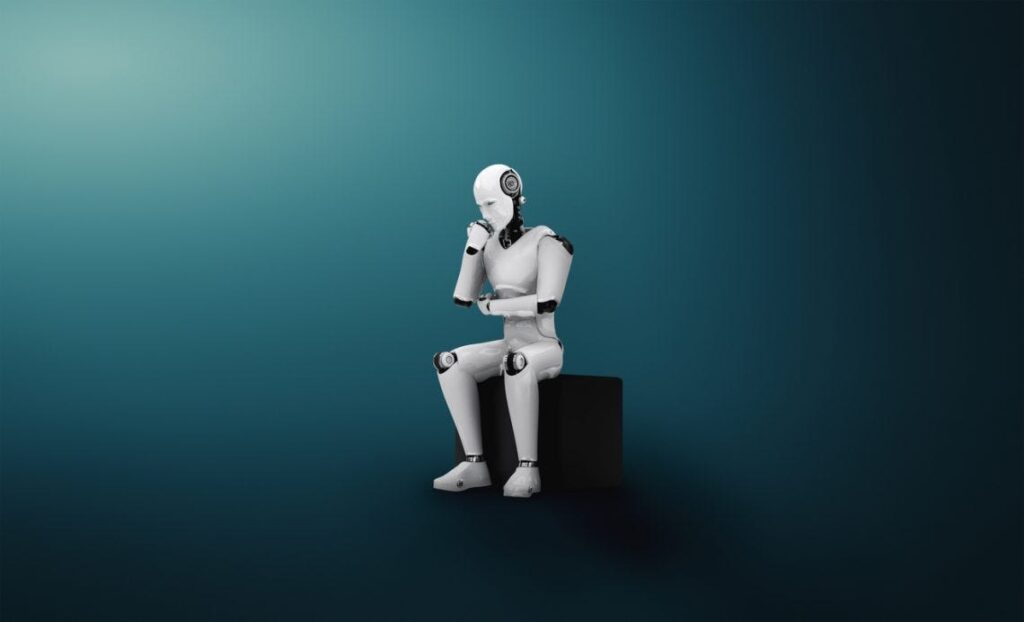
From image recognition to autonomous vehicles to predictive analytics in healthcare, artificial intelligence (AI) applications are exploding today. Taking a conscious look at the methodology of AI, we discover that the development of an AI application involves the acquisition of a large amount of data and the creation of various data sets for training, testing, and evaluation, and then the deployment of the application. Throughout this process, data and data labeling companies play an important role at every stage as it is essential that successive rounds of training, testing, and evaluation are conducted until the desired outcome is achieved.
Significance of data for developing AI models
Creating the appropriate datasets and data pipelines to develop and evaluate AI models is increasingly the biggest challenge. It is imperative that data labeling companies are involved in the process of developing artificial intelligence algorithms, as without them, access to the data (which makes the ML model learn, interpret, and act) is hindered. Having access to accurate and relevant data is essential when it comes to training AI systems as well as refining and improving their performance.
The role of data in AI development is important at every stage. A system’s structure and architecture are determined by data during the design phase. The problem to be solved as well as the data types to be used need to be understood. Other key steps involved in developing a full-fledged AI application are as follows:
- The AI system is trained based on the data collected after the design has been completed. To refine its algorithms and improve its performance, the system relies on large amounts of data. In addition to databases, text documents, images, and videos, data can also be derived from various sources.
- Data is used to evaluate the performance of the AI system once it has been trained. This is accomplished by testing and providing feedback on the system’s performance on a variety of tasks. In order to improve the system, the algorithms are further refined based on this feedback.
- The AI system is deployed with the help of data. To ensure that the system is working properly, real-world scenarios are used to test it. To ensure that the system continues to function correctly, the system is also monitored over time using data.
The use of artificial intelligence algorithms in conjunction with data allows them to analyze and identify patterns, correlate data, develop insights and solutions, and predict outcomes. AI systems can make decisions and take actions based on data that are generated through the use of models as data helps the model continuously learn and adapt to changing environmental conditions.
Role of data labeling companies in AI development
With data being a crucial component of AI development, the role of data labeling companies has become increasingly important, as they facilitate the access and utilization of data for AI developers. In any AI project, data labelers are responsible for ensuring that the datasets are accurate, current, and consistent. In order for AI models and applications to be reliable and accurate, this is imperative.
Creating datasets for training AI models/applications begins with the accurate labeling of data. When data is appropriately labeled, it is made more useful for AI development through the addition of relevant labels, such as text tags, image annotations, and 3D object recognition. These tags added to the data provide additional context to data through the use of semantic algorithms. In addition to providing an additional layer of analysis, they also ensure that the data is secure and that it complies with data privacy laws.
Assessing the availability of data for AI model
The availability of data for AI depends on the type of AI being used. For example, supervised learning requires labeled data sets, which are often provided by businesses or research groups for specific tasks. Unsupervised learning, however, requires large amounts of unlabeled data, which can be more difficult to obtain. Additionally, data for AI must be relevant and timely, as AI algorithms are only as effective as the data they are trained on. Finally, data must be collected, stored, and maintained in a secure manner to protect the privacy and comply with legal and ethical requirements.
Collecting data for training AI
The collection of data is a critical element of AI and machine learning, and a data labeling company has an extremely important role to play in ensuring the correct data is collected. It is the input that will be utilized to drive the algorithms that drive artificial intelligence. Data is essential to the learning and decision-making processes of the AI system. It is therefore important to collect high-quality data that is relevant to the AI project.
Data collection begins with determining the type of data required. It is possible for an AI project to include either structured or unstructured data, text, images, audio, or video, depending on the type of project. Additionally, it is important to consider the format of the data, such as CSV, JSON, or XML. Having identified the type of data requirement, one can begin with the data collection process.
Sourcing and cleaning data
There are many sources for obtaining data for AI development, which range from public databases, web APIs, and user-generated content. Importantly, the data collected should be relevant to the project. As an example, the data should include examples of fraudulent activity when the AI system is designed to detect fraud. In addition, it is critical to ensure that the data is properly documented and labeled. As a result, machine learning algorithms will be able to interpret and utilize the data more easily.
AI systems cannot learn and make decisions without high-quality, relevant data. Consequently, it is essential to ensure that the collected data is of the highest quality and properly documented and labeled. Data should be cleaned as soon as it is collected to ensure its quality and accuracy by removing duplicates and correcting typos. The AI system can begin using the data as soon as it has been cleaned.
Final Thought
Data accuracy is essential for AI to be reliable and effective. In order to train an AI model, AI systems rely heavily on data, and thus on data labeling companies for seamless access to accurate data. For that reason, it is imperative that the data used to train the AI model is accurate and up-to-date.
A system using AI can make incorrect or inefficient decisions if the data it uses is inaccurate or outdated. AI systems that are based on accurate data perform better, generate more accurate results, and prove to be more successful.