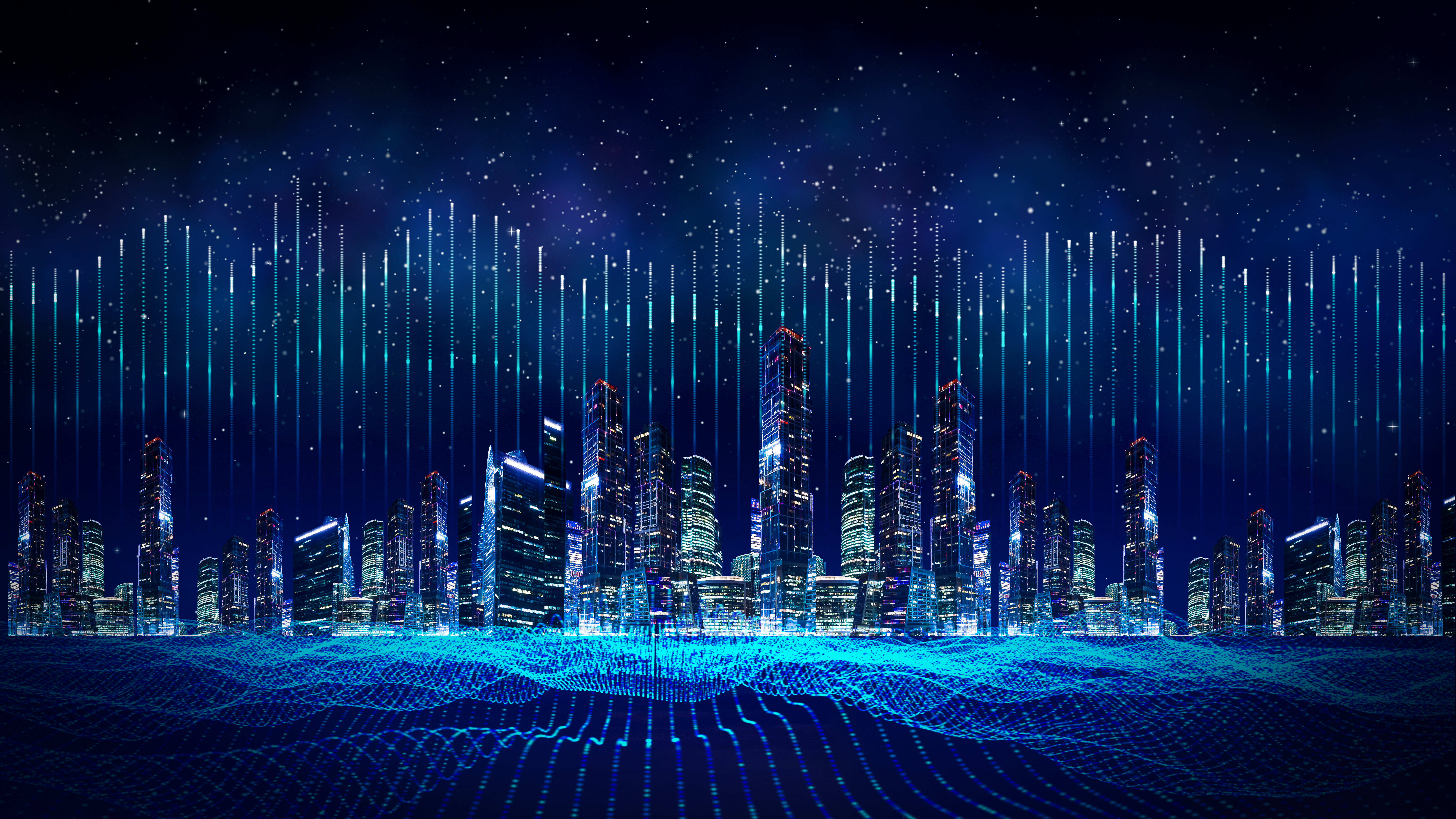
When an enterprise project is low-profile (“below the radar”), then it is not likely to be the target of bad actors. Similarly, if some part of that project’s infrastructure fails or falters, then the consequences of the problem and/or the urgency of providing a solution are usually manageable. But when a high-profile (“above the radar”) enterprise project goes wrong, then we must wrestle with these realities:
- Reviewing the “who, how, and why” of the failure is not a conversation that anyone wants to have.
- Resilience should have been a must-have feature of the infrastructure from the start.
- Rapid remediation (perhaps automated remediation) becomes a hard requirement.
- Root cause analysis (reactive, descriptive, diagnostic analytics) is exposed as a much less desirable activity when measured against the ROI of prescriptive interventions (optimizing, predictive reactive, early-warning precursor analytics).
What could be of higher profile (more “above the radar”) this year than Artificial Intelligence (AI), including generative AI and ChatGPT deployments in the enterprise? Nearly everyone is talking about it. Nearly every enterprise is already either planning, deploying, or running an AI project on some AI infrastructure. Along with all the goodness that this promises to bring, there is also the badness. Just recently, it was reported that login credentials have been stolen for over 100,000 hacked ChatGPT accounts and have appeared on dark web marketplaces. (REF1) While a rapidly increasing number of organizations are deploying AI in enterprise projects, in cybersecurity operations, and in other enterprise IT applications, AI is also being increasingly used by cyber infrastructure attackers. (REF2)
When I first heard about AIOps, I assumed it was similar to DevOps, MLOps, and DataOps – which are systems approaches to the efficient development and deployment of IT software operations, machine learning operations, and data operations, respectively. In other words, I thought that AIOps must be a similar systems approach to development and deployment of AI operations. I was so convinced of this interpretation that I might even have given a lecture or two on that topic at that time.
As interesting as my interpretation of AIOps might have been (to me, at least), I found the actual meaning of AIOps to be even more interesting. Specifically, AIOps refers to AI for IT operations. If I was responsible for naming it, I might have called it AITOps, sort of like DevSecOps (the practice of integrating security testing into the software development process) or like AIoT – the latter being AI for IoT (Internet of Things) operations.
What is AIOps and why do I now consider it to be so very interesting to a data scientist? Before I answer that, I must admit that my initial reaction to “AI for IT operations” was “oh, this is an I.T. infrastructure function, thus not relevant to a data scientist like me.” How wrong I was!
AIOps is a technology-driven approach that combines AI and machine learning (ML) techniques with traditional IT operations to enhance and automate various aspects of IT management and monitoring. Automation – I like that – check! Monitoring – I really like that – double-check!
Both automation and monitoring are data-fueled, data-powered, data-enabled, and generate business value from data – they are all about that data! That’s definitely within my definition of cool data stuff.
AIOps leverages ML and AI to analyze vast amounts of data generated by IT systems, networks, and applications. By sensing, monitoring, capturing, and modeling the patterns in data flows, data scientists are able to provide real-time insights, predictive (precursor) analytics, and automated prescriptive responses to many diverse business operations. So, why would the sphere of data scientists’ activities exclude IT operations? It doesn’t, of course! Why? Because… AIOps can improve the efficiency, reliability, and resilience of IT operations by enabling these use cases: proactive problem detection, faster incident response, and intelligent decision-making. All of those use cases are (generally) analogous to other business use cases powered by data (customers, sales, supply chain, digital assets, HR, finance, etc.) in which data scientists are already engaged.
Further underscoring the interestingness of AIOps to a data scientist is the key fact that AIOps utilizes advanced algorithms and models to process, analyze, interpret, and derive inferences from massive volumes of data from diverse sources, such as log files, monitoring tools, event streams, and performance metrics. In reference to diverse data sources, I have always said that “variety is the spice of discovery”. Exploring high-dimensional (diverse, high-variety) data can lead to deeper insights (the “360 view”), discovery of hidden patterns (non-differentiated in low-dimensional data projections), uncovering proof of causal relationships, and far less modeling bias than using single data sources. Those prospects are very appealing to this data scientist.
By applying AI and ML techniques that can identify patterns, anomalies, associations, correlations, and causal connections within the data, IT teams gain a deeper visibility into the performance and health of their IT infrastructure. AIOps platforms can automatically detect and prioritize critical issues, generate actionable insights, and even predict potential problems before they occur. This enables IT teams to take proactive measures, reduce downtime, and optimize resource allocation.
Furthermore, AIOps helps streamline IT operations by automating routine tasks and workflows. Through intelligent automation, AIOps can handle repetitive and time-consuming activities such as event correlation, root cause analysis, and remediation actions. By leveraging AI-powered automation, organizations can significantly improve their operational efficiency, reduce human error, and allocate resources to more strategic initiatives. AIOps also facilitates collaboration and communication among different IT teams by providing a centralized platform that consolidates data, insights, and workflows, thereby enabling faster decision-making and effective problem resolution.
Automated remediation (including anomaly detection, prescriptive optimizations, and incident response) is a feature of a general concept that I discussed in earlier publications: “Safe Driving in the Self-Driving Enterprise”. (REF 3)
Maybe AIOps really is about AI operations as I thought at the beginning – securing, governing, and monitoring the data flows that power AI is a critical AIOps function. That’s an enterprise-worthy example of using AI to keep an eye on the AI – i.e., monitoring and analyzing the data generated by IT systems, networks, and applications to keep the AI secure, trusted, performant, and optimized. Consequently, AIOps tools, techniques, and applications should employ AI for more than observability / monitoring / alerting / risk management of the IT and network infrastructure. AIOps should also employ the AI to key an eye on the enterprise AI deployments. That includes full-spectrum analytics “above the radar”: descriptive, diagnostic, predictive, and prescriptive – all the flavors of modeling and analytics to fully engage the data science team.
REFERENCES:
(REF 1) https://thehackernews.com/2023/06/over-100000-stolen-chatgpt-account.html
(REF 3) https://medium.com/@kirk.borne/safe-driving-in-the-self-driving-enterprise-656fd3bbf378
(REF 4) https://www.techtarget.com/searchitoperations/definition/AIOps (source for graphic)
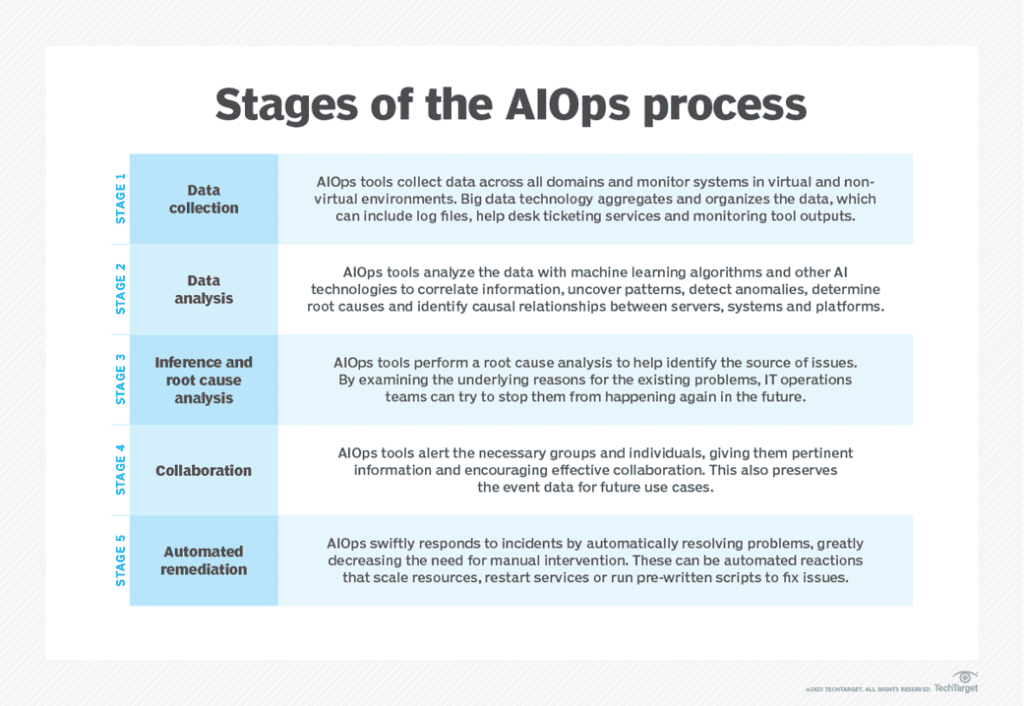
(Source: https://www.techtarget.com/searchitoperations/definition/AIOps)