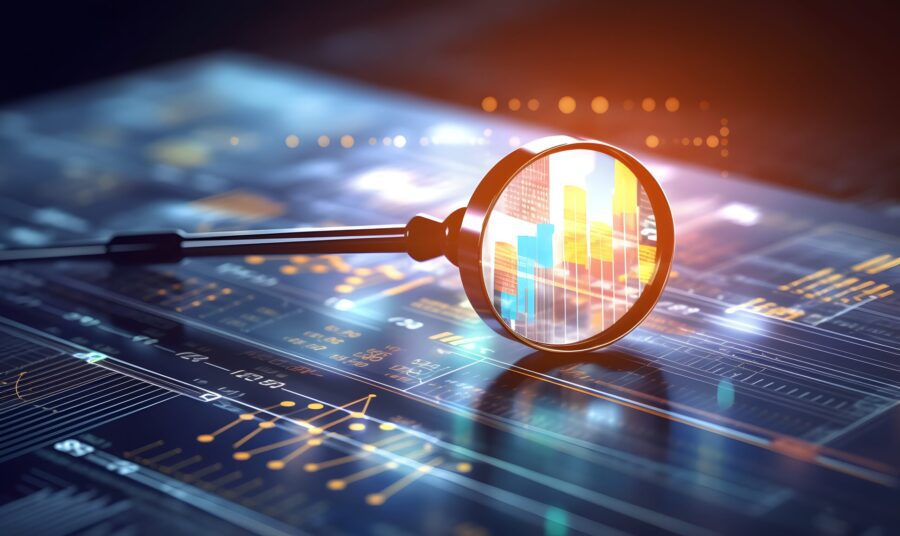
The 21st century has seen progress in technology with artificial intelligence (AI) making an impact across various industries, including finance. The potential benefits of AI such as efficiency, better decision making, and cost reduction have generated interest in its adoption within the financial sector. Despite these advantages, there are challenges that hinder the seamless integration of AI into financial services. In this article, we will explore five obstacles that impede the implementation of AI in finance and provide valuable insights and practical tips to overcome them.
1. Balancing Innovation and Compliance; Navigating Regulatory Complexities
One of the hurdles faced by the financial services industry when embracing AI is navigating through regulatory landscapes. Financial institutions operate within a regulated environment to ensure consumer protection, data privacy and fair practices. Integrating AI into this framework requires a delicate balance between embracing innovation and ensuring compliance with stringent regulations.
Actionable Insights:
- Stay Informed: Stay updated on evolving regulations specific to AI and finance, such as GDPR, CCPA, and sector-specific guidelines. Collaborate with legal experts to navigate the regulatory landscape effectively.
- Transparency: Implement AI solutions that offer transparency into decision-making processes. This not only aids in building trust with regulators but also helps explain AI-driven decisions to customers.
2. Data Security and Privacy Concerns: Safeguarding Sensitive Information
AI heavily relies on data to generate insights and predictions. In a sector where safeguarding customer information’s critical concerns about data security and privacy pose significant barriers to AI adoption. The potential risks associated with data breaches, unauthorized access to information or misuse of customer data can have consequences.
Actionable Insights:
- Robust Encryption: Employ strong encryption methods to secure data both in transit and at rest. This ensures that even if data is compromised, it remains indecipherable without proper authorization.
- Access Controls: Implement stringent access controls to limit data access to authorized personnel only. Multi-factor authentication and role-based access can bolster data protection.
- Anonymization: When possible, work with anonymized or pseudonymized data for AI model training. This reduces the risk of exposing personally identifiable information.
3. Lack of Quality Data: The Foundation of Effective AI
AI algorithms thrive on high-quality, diverse, and representative data. In the financial realm, acquiring such data can be challenging due to its complex and dynamic nature. Inaccuracies and biases in the data can lead to flawed AI models, hindering accurate predictions and decisions.
Actionable Insights:
- Data Preprocessing: Invest in robust data preprocessing techniques to cleanse, normalize, and transform raw data. This enhances the quality and reliability of the data used for AI training.
- Data Augmentation: Augment existing datasets with simulated data to fill gaps and improve the diversity of training data.
- Continuous Monitoring: Establish processes to continuously monitor data quality and update models as new, accurate data becomes available.
4. Resistance to Change: Overcoming Organizational Inertia
Implementing AI in finance often necessitates a cultural shift within organizations. Resistance to change, fear of job displacement, and lack of understanding about AI’s potential benefits can hinder adoption efforts.
Actionable Insights:
- Education and Training: Offer comprehensive training programs to familiarize employees with AI concepts, its advantages, and its limitations. This empowers them to embrace AI as a tool rather than a threat.
- Clear Communication: Transparently communicate the objectives and benefits of AI adoption. Address concerns and provide a clear roadmap for how AI will complement existing roles.
- Incentives: Introduce incentives to encourage employees to engage with AI initiatives actively. Recognize and reward contributions toward successful AI integration.
5. Cost and Resource Constraints: Making AI Investment Feasible
While the potential long-term benefits of AI adoption in financial services are substantial, the initial investment can be a deterrent. AI implementation requires significant financial resources, including infrastructure, talent acquisition, and ongoing maintenance.
Actionable Insights:
- Pilot Projects: Start with small-scale pilot projects to demonstrate AI’s value without committing to large expenditures upfront.
- Cloud Services: Consider leveraging cloud-based AI services and platforms to reduce the need for extensive hardware investments.
- Collaboration: Explore partnerships with fintech startups or AI service providers to share costs and access specialized expertise.
Conclusion
AI’s transformative potential in financial services is undeniable, but its widespread adoption faces several obstacles. Navigating regulatory challenges, prioritizing data security, ensuring data quality, overcoming resistance to change, and managing costs are critical steps for successful integration. By addressing these obstacles with the actionable insights provided, financial institutions can pave the way for a future where AI-driven advancements reshape the landscape of financial services.
FAQs:
Q1: How is AI currently used in the financial industry?
AI is used in the financial industry for a range of applications, including fraud detection, credit risk assessment, algorithmic trading, customer service chatbots, and personalized financial advice. These applications leverage AI’s ability to analyze vast amounts of data and make predictions or decisions in real-time.
Q2: What are the potential benefits of AI adoption in financial services?
AI adoption in financial services can lead to enhanced efficiency, improved decision-making accuracy, cost reduction, and the ability to offer more personalized services to customers. AI can also automate time-consuming tasks, allowing employees to focus on higher-value strategic activities. Additionally, it can help identify patterns and trends in financial data that may not be apparent through traditional analysis methods, enabling better risk management and investment strategies.
Q3: Are there any ethical concerns associated with AI in finance?
Yes, there are ethical concerns related to AI in finance. These include issues like biased algorithms that may discriminate against certain groups, lack of transparency in AI decision-making processes, and concerns about data privacy and security. Regulators and industry stakeholders are actively working to address these ethical challenges in the use of AI in finance.
Q4: How can professionals develop AI skills for a career in finance?
Professionals interested in AI in finance can start by taking online courses and certifications in machine learning, data analytics in investment banking, and AI technologies. Additionally, they can engage in projects or internships that involve AI applications in financial services to gain practical experience. Staying updated with industry trends and networking with professionals in the field can also be valuable for skill development and career advancement.