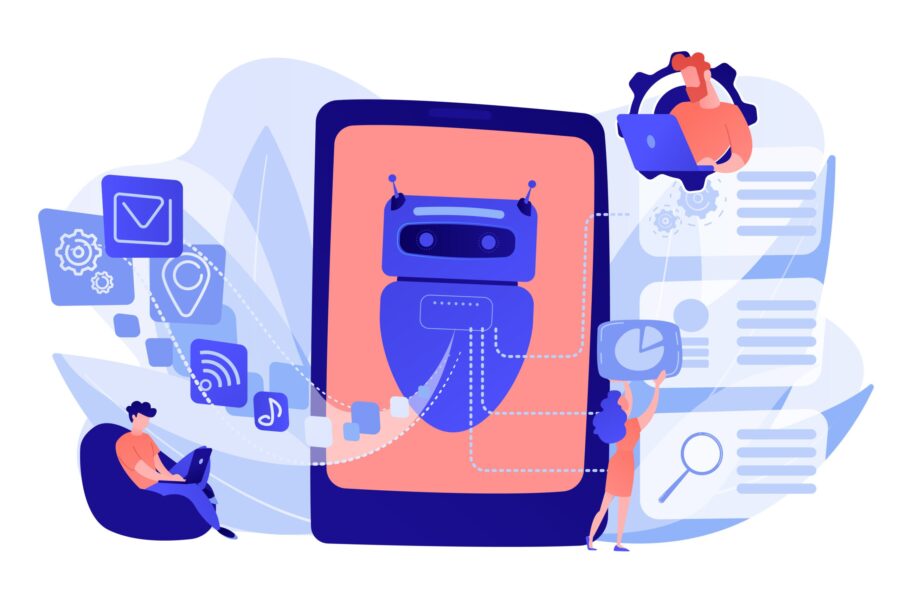
In the panorama of Artificial Intelligence (AI), Natural Language Understanding (NLU) stands as a citadel of computational wizardry. No longer in its nascent stage, NLU has matured into an irreplaceable asset for business intelligence. In this discussion, we delve into the advanced realms of NLU, unraveling its role in semantic comprehension, intent classification, and context-aware decision-making.
The underpinnings: Language models and deep learning
Before embarking on the NLU journey, distinguishing between Natural Language Processing (NLP) and NLU is essential. While NLP is an overarching field encompassing a myriad of language-related tasks, NLU is laser-focused on understanding the semantic meaning of human language.
The backbone of modern NLU systems lies in deep learning algorithms, particularly neural networks. These models, such as Transformer architectures, parse through layers of data to distill semantic essence, encapsulating it in latent variables that are interpretable by machines. Unlike shallow algorithms, deep learning models probe into intricate relationships between words, clauses, and even sentences, constructing a semantic mesh that is invaluable for businesses.
Transformers and attention mechanisms
In advanced NLU, the advent of Transformer architectures has been revolutionary. These models leverage attention mechanisms to weigh the importance of different sentence parts differently, thereby mimicking how humans focus on specific words when understanding language. For instance, in sentiment analysis models for customer reviews, attention mechanisms can guide the model to focus on adjectives such as ‘excellent’ or ‘poor,’ thereby producing more accurate assessments.
Semantic eearch in customer service
Semantic search capabilities have revolutionized customer service experiences. NLU algorithms sift through vast repositories of FAQs and support documents to retrieve answers that are not just keyword-based but contextually relevant. What sets semantic search apart is its ability to consider user intent. By employing semantic similarity metrics and concept embeddings, businesses can map customer queries to the most relevant documents in their database, thereby delivering pinpoint solutions.
Concept embeddings and knowledge graphs
It would be remiss to ignore the role of concept embeddings and knowledge graphs when talking about semantic search. These technologies allow NLU algorithms to map abstract concepts to vectors in a high-dimensional space, facilitating better search outcomes. For instance, customer inquiries related to ‘software crashes’ could also yield results that involve ‘system instability,’ thanks to the semantic richness of the underlying knowledge graph.
Sentiment analysis for market research
One of the most compelling applications of NLU in B2B spaces is sentiment analysis. Utilizing deep learning algorithms, businesses can comb through social media, news articles, & customer reviews to gauge public sentiment about a product or a brand. But advanced NLU takes this further by dissecting the tonal subtleties that often go unnoticed in conventional sentiment analysis algorithms.
Multi-dimensional sentiment metrics
In sentiment analysis, multi-dimensional sentiment metrics offer an unprecedented depth of understanding that transcends the rudimentary classifications of positive, negative, or neutral feelings. Traditional sentiment analysis tools have limitations, often glossing over the intricate spectrum of human emotions and reducing them to overly simplistic categories. While such approaches may offer a general overview, they miss the finer textures of consumer sentiment, potentially leading to misinformed strategies and lost business opportunities.
On the other hand, multi-dimensional sentiment metrics delve into the complexity of human emotions by capturing a range of nuanced sentiments such as ‘enthusiasm,’ ‘skepticism,’ or ‘indifference.’ This approach allows businesses to identify subtler emotional currents within customer interactions, social media engagements, or product reviews. For example, a consumer may express skepticism about the cost-effectiveness of a product but show enthusiasm about its innovative features. Traditional sentiment analysis tools would struggle to capture this dichotomy, but multi-dimensional metrics can dissect these overlapping sentiments more precisely.
Granular sentiments
The value of understanding these granular sentiments cannot be overstated, especially in a competitive business landscape. Armed with this rich emotional data, businesses can finetune their product offerings, customer service, and marketing strategies to resonate with the intricacies of consumer emotions. For instance, identifying a predominant sentiment of ‘indifference’ could prompt a company to reinvigorate its marketing campaigns to generate more excitement. At the same time, a surge in ‘enthusiasm’ could signal the right moment to launch a new product feature or service.
This level of specificity in understanding consumer sentiment gives businesses a critical advantage. They can tailor their market strategies based on what a segment of their audience is talking about and precisely how they feel about it. The strategic implications are far-reaching, from product development to customer engagement to competitive positioning. Essentially, multi-dimensional sentiment metrics enable businesses to adapt to shifting emotional landscapes, thereby crafting strategies that are responsive and predictive of consumer behavior. Therefore, companies that leverage these advanced analytical tools effectively position themselves at the forefront of market trends, gaining a competitive edge that is both data-driven and emotionally attuned.
Also Read: How Businesses can benefit by Integrating ChatGPT in their Apps
Anomaly detection in textual data
Another groundbreaking application is anomaly detection within textual data. Conventional techniques often falter when handling the complexities of human language. Enter NLU. By mapping textual information to semantic spaces, NLU algorithms can identify outliers in datasets, such as fraudulent activities or compliance violations.
Semantic hashing and information retrieval
Techniques like semantic hashing are instrumental in these efforts. These algorithms can swiftly perform comparisons and flag anomalies by converting textual descriptions into compressed semantic fingerprints. This is particularly beneficial in regulatory compliance monitoring, where NLU can autonomously review contracts and flag clauses that violate norms.
Ethical implications: NLU and data privacy
As with any technology, the rise of NLU brings about ethical considerations, primarily concerning data privacy and security. Businesses leveraging NLU algorithms for data analysis must ensure customer information is anonymized and encrypted.
Secure multi-party computation
In this regard, secure multi-party computation techniques come to the forefront. These algorithms allow NLU models to learn from encrypted data, ensuring that sensitive information is not exposed during the analysis. Adopting such ethical practices is a legal mandate and crucial for building trust with stakeholders.
Conclusion: The future is semantic
As AI development continues to evolve, the role of NLU in understanding the nuanced layers of human language becomes even more pronounced. From semantic search in customer service to multi-dimensional sentiment analysis in market research, the applications are manifold and invaluable for B2B ventures.
It’s abundantly clear that NLU transcends mere keyword recognition, venturing into semantic comprehension and context-aware decision-making. As we propel into an era governed by data, the businesses that will stand the test of time invest in advanced NLU technologies, thereby pioneering a new paradigm of computational semiotics in business intelligence.