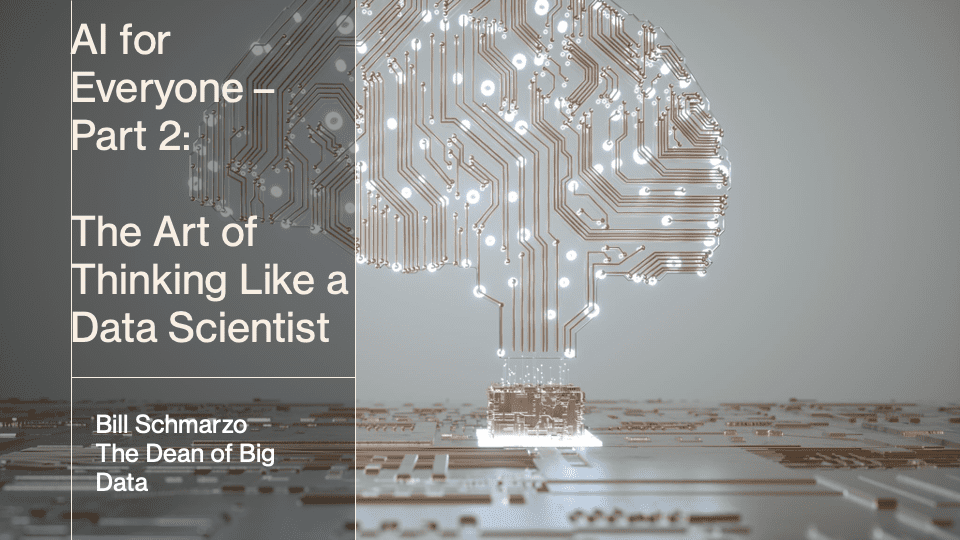
In Part 1 of the series “AI for Everyone: Learn How to Think Like a Data Scientist”, we discussed that for AI to reach its full economic and societal potential, we must educate and empower everyone to actively participate in the design, application, and management of meaningful, relevant, and responsible AI. We discussed the role that the “Thinking Like a Data Scientist” methodology can play in driving an inclusive, collaborative process that empowers everyone to participate in defining the variables and metrics against which organizations define and measure their value creation effectiveness.
We also introduced an “AI for Everyone” playbook that outlines the role that everyone needs to play in delivering responsible and ethical AI models. In Part 1, we started with the following steps:
- Step 1) Defining Value
- Step 2) Understanding How AI Works
- Step 3) Understanding the Role of the AI Utility Function
Now, let’s cover the rest of the “AI for Everyone” playbook.
Step 4) Building a Healthy AI Utility Function
Defining variables and metrics that comprise AI Utility Function too narrowly can lead to AI model confirmation bias and potentially devastating unintended consequences.
As we define the variables and metrics against which we want the AI Utility Function to optimize, we must embrace an expanded view of how value is defined and created. That means moving beyond the traditional and operational metrics against which most organizations seek to optimize with their advanced analytics.
Remember, AI is a learning tool, and one must broaden the dimensions against which value creation is defined and measured. That means including variables and metrics related to customer satisfaction and advocacy, employee satisfaction and development, partner ecosystem financial and economic viability, environmental conditions, diversity and social factors, and ethical “do good” factors (Figure 1).
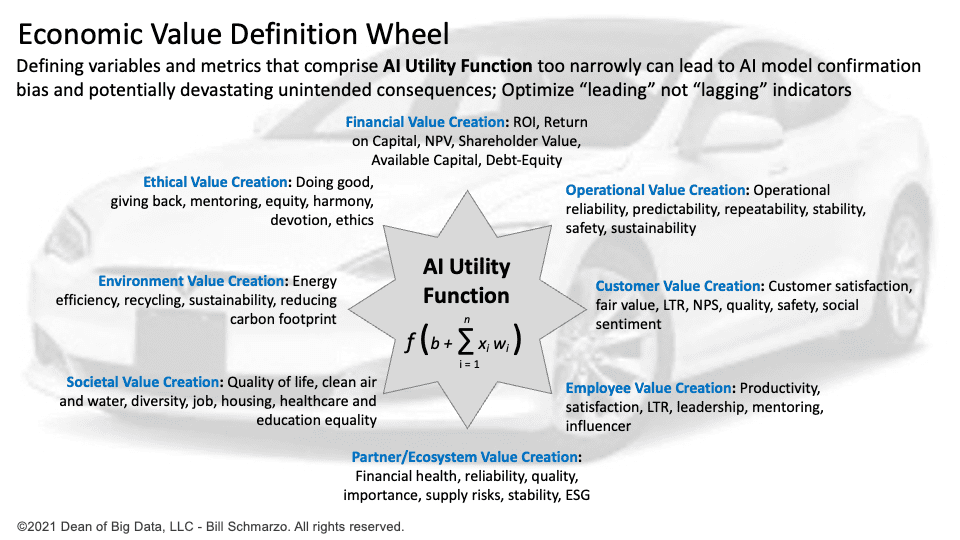
Figure 1: Economic Value Definition Wheel
Remember, only defining financial metrics will lead to AI model confirmation bias and a gradually shrinking total addressable market. And only defining lagging indicators will only lead to potentially dangerous unintended consequences.
Step 5) Building Conflict Into the AI Utility Function
Multi-objective Optimization is a branch of decision-making that deals with mathematical optimization problems requiring simultaneous optimization of multiple objective functions.
AI models will access, analyze, and make decisions billions, if not trillions, of times faster than humans. An AI model will find the gaps in a poorly constructed AI Utility Function and will quickly exploit that gap to the potential detriment of many key stakeholders. Consequently, build conflicting variables and metrics into the AI Utility Function – Improve A while reducing B, improve C while also improving D.
Just like humans are constantly forced to make difficult trade-off decisions (i.e., drive to work quickly but also arrive safely, improve the quality of healthcare while improving the economy), we must expect nothing less from a tool like AI that can make those tough trade-off decisions without biases or prejudices. The good news is that several multi-objective optimization algorithms excel at optimizing multiple conflicting objectives (Figure 2).
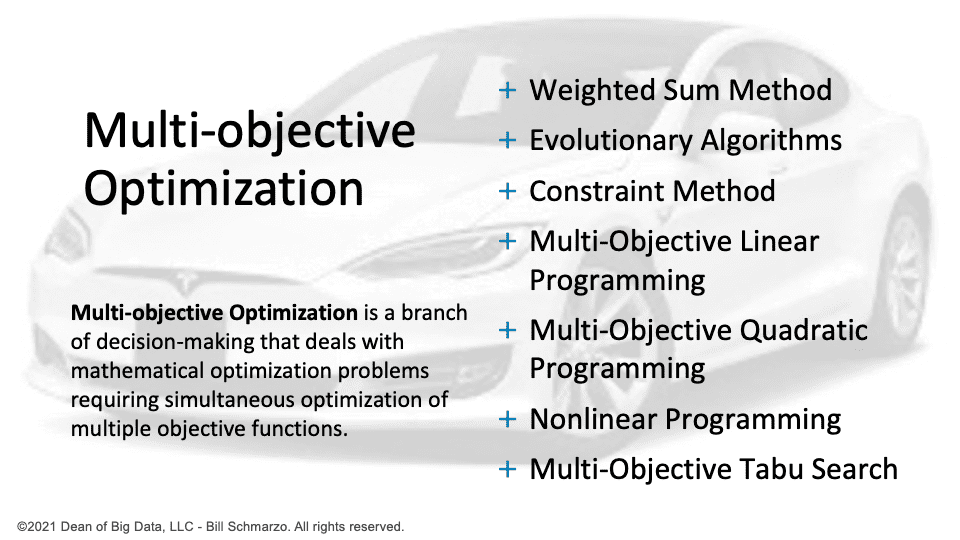
Figure 2: Multi-objective Optimization Algorithms
AI for Everyone Summary
If we want AI to work for the benefit of society, then we must prepare and empower everyone to actively participate. That means both demystifying or simplifying what and how AI works and educating and empowering everyone as to their role in ensuring the design, development, deployment, and ongoing management of the AI models that work for the benefit of humanity. Understanding the “Thinking Like a Data Scientist” methodology is a simple starting point (Figure 3).
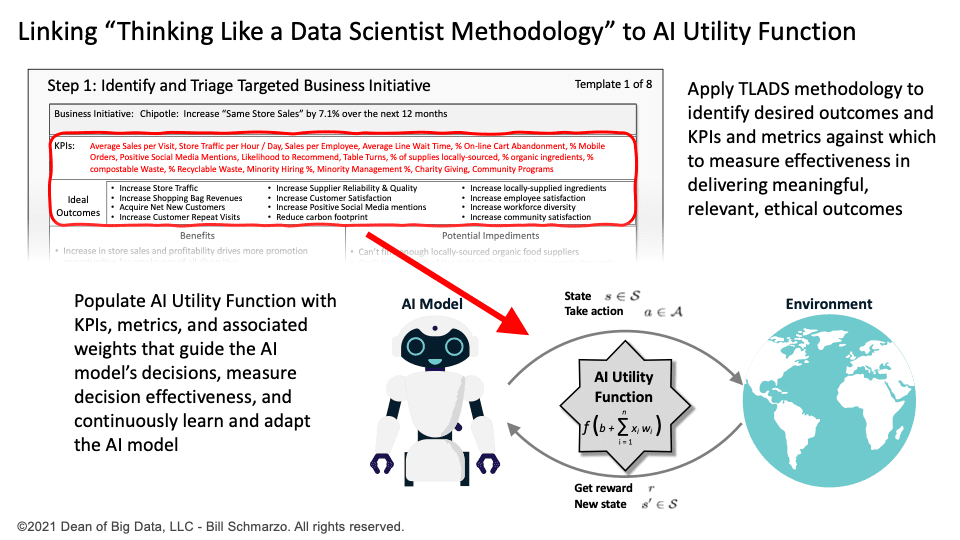
Figure 3: Linking Thinking Like a Data Scientist Methodology to the AI Utility Function
The AI for Everyone playbook ensures that everyone understands their role – and their responsibility – in developing responsible and ethical AI models. There is no value in whining about not being included in the conversation when the playbook is right in front of you.
By the way, I was curious about what ChatGPT thought about the relationship between my Thinking Like a Data Scientist methodology and creating a healthy AI Utility Function (Figure 4).
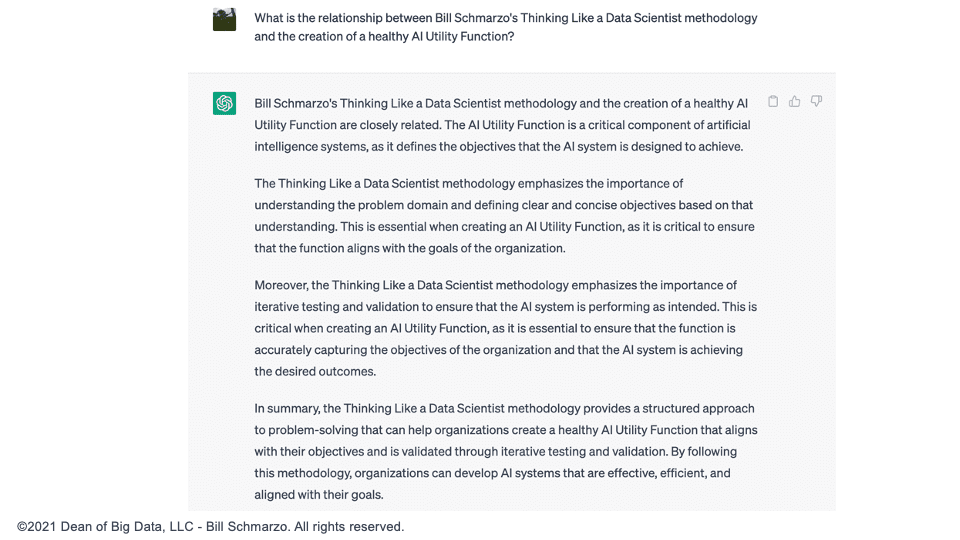
Figure 4: ChatGPT’s Assessment of the Relationship Between TLADS Methodology and AI Utility Function
Well, I know someone who will get an “A” in my class. Teacher’s pet…