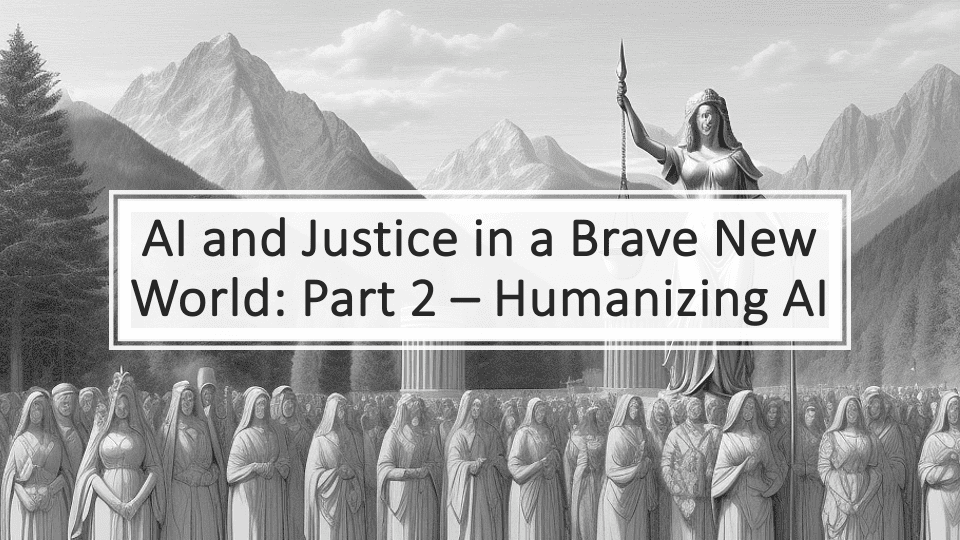
In part 1 of the series “A Different AI Scenario: AI and Justice in a Brave New World,” we outlined some requirements for the role that AI would play in enforcing our laws and regulations in a more just and fair manner and what our human legislators must do to ensure those more just and fair outcomes.
Let’s look at a real example of how AI is being used to enforce our laws and regulations in a more unbiased, consistent, and transparent manner…robot umpires.
Rise of the Robot Umpires
Baseball has always been an excellent testing ground for analytics (Strat-o-matic baseball, Sabermetrics, Money Ball, etc.). While baseball rules are clearly defined, enforcing them has always been left to humans. And the inconsistent and obscure enforcement of those baseball rules is being challenged.
In 2018, Major League Baseball (MLB) professional umpires missed 34,294 strike zone calls. That same year, 55 games were ended on incorrect calls by umpires[1]. The inconsistent enforcement of the strike zone impacts the fortunes of teams and players in a sport where teams are worth tens of billions of dollars and the top players earn nearly a quarter of a billion dollars.
The challenge is that human umpires are…well, human. And humans have ingrained biases and prejudices that are put on full display when that human stands behind home plate in a professional baseball game and must distinguish between balls and strikes in a fraction of a second (Figure 1).
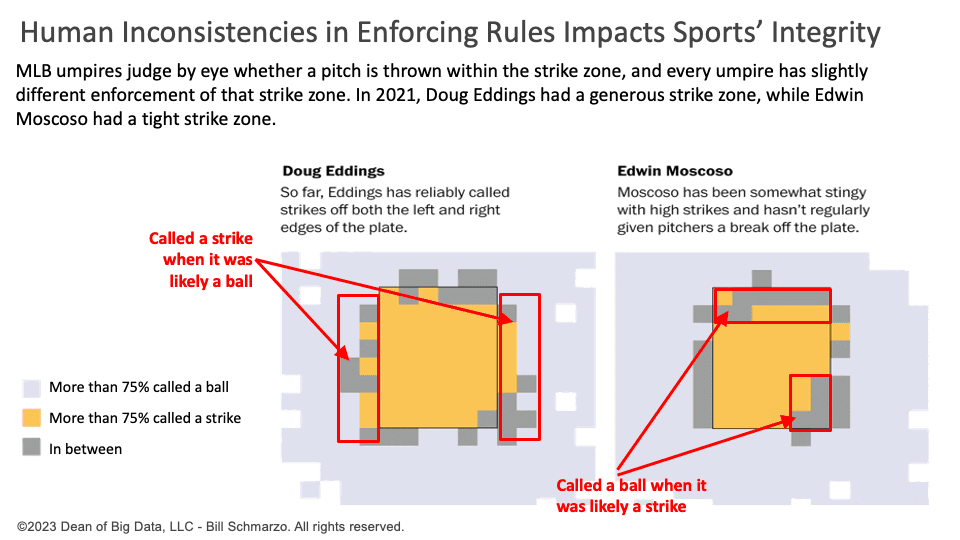
Figure 1: Human Inconsistencies in Enforcing Rules Impacts the Sports Integrity
Enter Robot umpires. A robot umpire is an automated system to call balls and strikes. The system uses a radar or a camera to track the location and trajectory of each pitch and compares it with the predefined strike zone calibrated for the physical dimensions of each batter. The system then communicates the call to the human umpire via an earpiece or a screen.
Some baseball purists are concerned that the robot umpire is too black and white and that the best human umpires consider specific nuances of the players before rendering their call. For example, a pitcher like Greg Maddux, with a history of outstanding pitching performance, has “earned the benefit” of a slightly larger strike zone. Or a Hall of Fame batter like Barry Bonds has “earned the benefit” of a smaller strike zone given his historical batting performance (Figure 2).
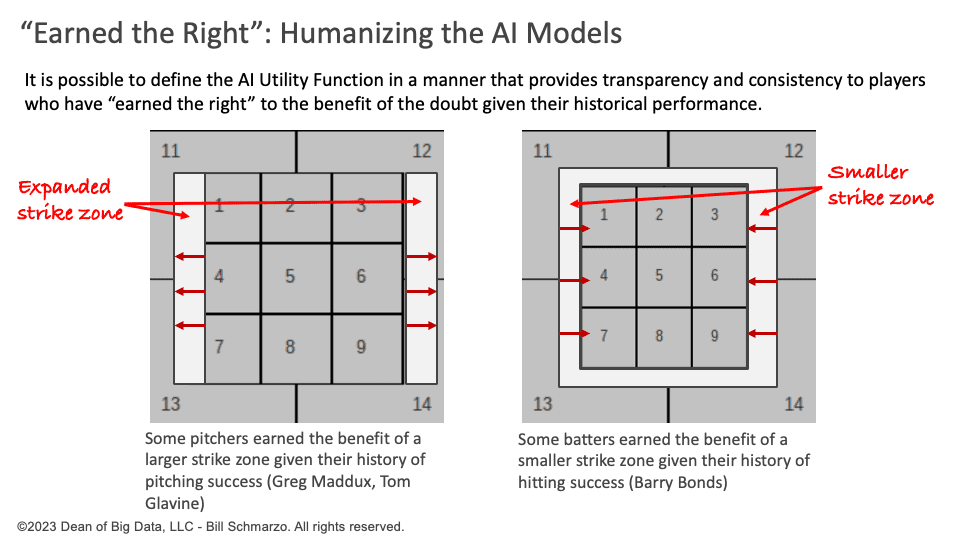
Figure 2: “Earned the Right” and Humanizing the AI Models
Adding these nuances to the enforcement of the strike zone is what makes humans human. But let’s decompose what “earned the right” really means:
- “Earned” means to whom special consideration is given in enforcing a rule given that person’s historical performance. “Earned” determines the “who” in rule enforcement leniency.
- “The Right” means how many degrees of latitude are given to that person when considering rule enforcement leniency; that is, the level of latitude from the defined rule the person is given based on their historical performance.
This “earned the right” concept of considering additional factors in enforcing a law or regulation can apply to several other scenarios, including:
- A driver may have “earned the right” for police officer leniency for a speeding ticket based on their driving record, safe driving behaviors, weather conditions, amount of traffic, time of day, etc.
- A customer may have “earned the right” for credit agent leniency for a credit card late fee charge based on their payment history, frequency of late payments, FICO score, lifetime value, etc.
- A student may have “earned the right” for teacher leniency to a student for missing a homework deadline based on their academic record, classroom workload, extracurricular activities, personal circumstances, attendance record, etc.
- An employee may have “earned the right” for manager leniency for an unexcused absence based upon their hardworking reputation, attendance record, performance reviews, positive organizational influence, outside volunteer efforts, etc.
- A state resident may have “earned the right” for DMV clerk leniency for not providing the proper documentation in renewing their driver’s license based on years of residence, on-time payment history, timely filing of paperwork, etc.
Yes, the AI model can be engineered to give people rule enforcement leniency based on their past performance. The key is that the criterion for receiving leniency is determined beforehand. And it is transparent why someone is given such leniency.
Legal Tests: Frye and Standard Daubert Standards
We can learn much from the legal community about the proper and thoughtful enforcement of laws and regulations. The legal community has decades of experience regarding the admissibility of evidence in legal enforcement cases. For example, the Frye standard is a judicial test used to determine the admissibility of scientific evidence based on the scientific community’s generally accepted scientific methods. And because the Frye standard has been criticized for being too rigid, many states and federal courts have adopted the Daubert standard, which considers other factors such as the scientific method’s testability, peer review, and error rate.
We could leverage the years of learning from applying the Frye and Daubert standards to select the variables and metrics that comprise a healthy, unbiased, fair AI Utility Function. For example, the Frye and Daubert standards could filter out variables and metrics in the AI Utility function that are not generally accepted by the relevant scientific community, testability, or peer review (Figure 3).
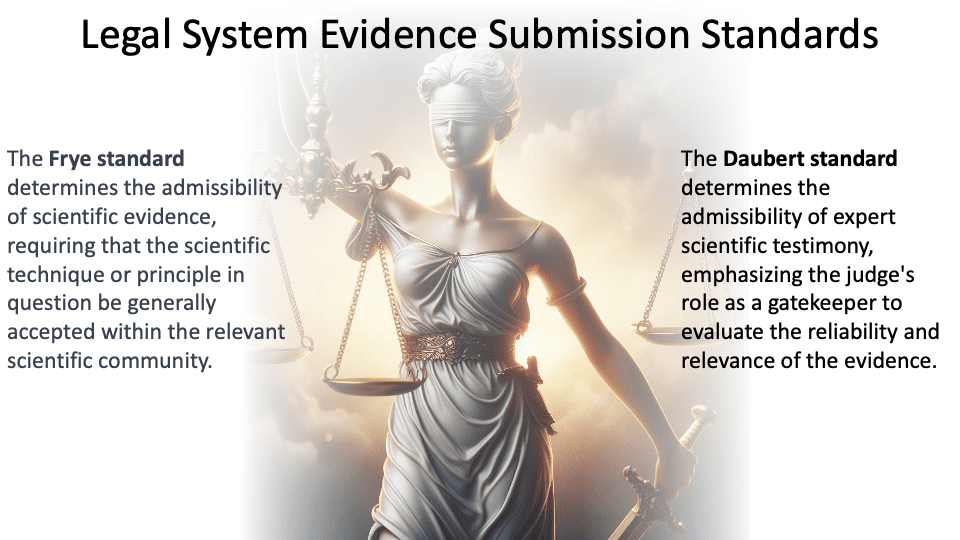
Figure 3: The Frye and Daubert Legal Admissibility Standards
The Frye, Daubert, and Kumho (another legal admissibility test) standards are judicial tests to determine the admissibility of scientific and expert evidence in enforcing laws and regulations. By integrating the learnings from applying these standards into the construction of the AI Utility Function and AI Certification models, we can learn how to balance simplicity, flexibility, transparency, and accountability in designing and evaluating AI models and their supporting AI Utility Function that enforce laws and regulations.
Summary: Create a More Fair, Just, and Empowered Society Part 2
As a society, we should aim to use AI to create a more fair and just world for everyone. This is an essential goal as we strive to leverage technology to improve the quality of life and opportunities for all, regardless of their background, status, or location. We must not allow this chance to be squandered or taken over by a privileged few with the resources and influence to exploit this pivotal moment for their selfish interests.
Part 3 will complete this blog series by discussing the importance of AI Governance and the role of an AI Model Audit Trail and AI certification engines to monitor and ensure unbiased, fair, and consistent application of AI.
[1] MLB Umpires Missed 34,294 Ball-Strike Calls in 2018. Bring on Robo-umps? https://www.bu.edu/articles/2019/mlb-umpires-strike-zone-accuracy/