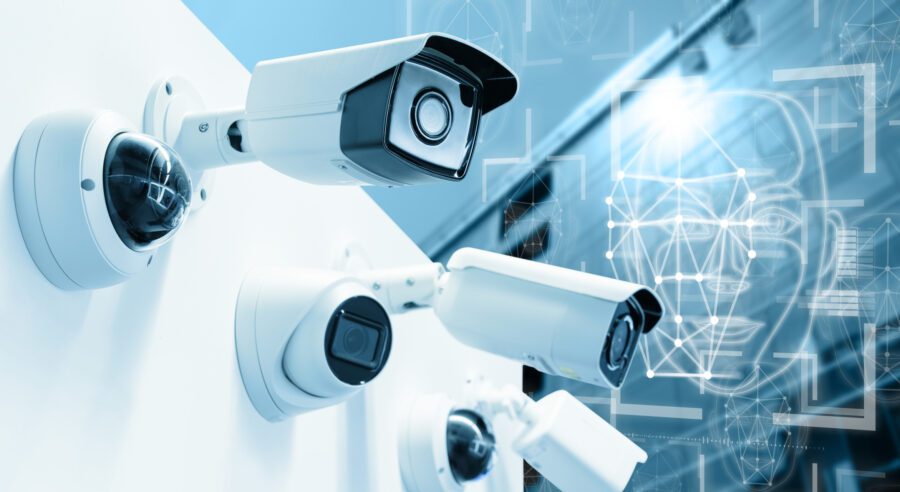
Artificial intelligence (AI) makes security cameras more versatile and useful. It can recognize suspicious behavior in real time, monitor video feeds to mitigate labor shortages and save clips of interest to streamline investigations. However, AI can also introduce or heighten surveillance concerns.
Organizations hoping to capitalize on the benefits of AI in closed-circuit television (CCTV) applications should consider its downsides. Only by addressing these areas can developers ensure their AI solution achieves its full potential.
1. Legal obstacles
Any CCTV system faces regulatory considerations, as where and how much a company can film varies depending on local laws. AI functions like facial recognition, potentially introducing legal concerns.
At least 15 states have facial recognition laws limiting the use of this technology. State legislatures also vary in how they approach biometric data collection, use and storage, which may fall under facial identification. Because these regulations are different in each area, determining what’s permissible and what isn’t can be a challenge.
AI security teams can start by researching their specific national, state and city laws. Turning to professional legal counsel may be necessary to avoid costly mistakes. When regulations are unclear, it’s safest to err on the side of caution, imposing more restrictions than may be required.
2. Ethical dilemmas
Similarly, the use of AI in video surveillance can raise ethical questions. While history shows that security cameras reduce crime rates, not everyone is comfortable with being watched. AI’s well-publicized issues with bias and false positive identifications push such concerns higher.
Implicit biases in training data can lead surveillance algorithms to reflect and exaggerate human prejudices. As a result, they may lead to even more unjust treatment of people of color. Even if no legal fallout occurs from an incident, companies must grapple with the social and ethical consequences.
Responsibility in training is the best way to tackle this issue. Using synthetic data instead of real-world information can help provide models with more diverse datasets to avoid biases. Regularly monitoring these systems for signs of prejudice and requiring teams to verify AI alerts before acting on them will also help.
3. Machine vision accuracy
AI’s accuracy and struggles with false positives can be concerning outside of ethical considerations. While some facial recognition algorithms have achieved accuracy levels over 90%, not all are so reliable.
It takes a considerable amount of data to ensure a machine vision model can effectively identify objects, people or other points of interest. Such information must also feature significant variation to prevent the overfitting that leads to false positives. Failing to supply enough data or use the right model will limit the solution’s ability to deliver real-world value.
Synthetic data can again help here, providing additional information for training a model without increasing security or bias risks. Thoroughly cleansing data before feeding it to the AI algorithm is also important. Hardware improvements can help, too, as a higher video resolution will make it easier for AI to identify objects of interest.
4. Data privacy
Even outside of training, AI video surveillance involves a substantial amount of data. The AI model saves clips for future investigations or to self-adapt over time, leading to a growing video data library. This could lead to massive breaches if a cybercriminal infiltrates the camera system and steals this information.
Facial recognition applications face the highest risks here, as criminals could use stolen biometric data to bypass security measures elsewhere or commit fraud. The businesses using these systems could face hefty penalties or reputational damage.
Given these risks, organizations must encrypt all video data and limit access to only professionals who need it for their jobs. Protecting such databases with multi-factor authentication and real-time automated monitoring is also crucial. Homomorphic encryption is worth considering, too, as it lets AI models learn from encrypted data without prior decryption.
5. Costs and complexity
As with other AI applications, smart video surveillance also introduces cost and technical complexity concerns. AI models are often expensive to build. Surveillance systems work best when integrating multiple Internet of Things (IoT) technologies, but this introduces more network complexity and costs.
Over time, increased efficiency should make up for these initial expenses. However, the high entry bar can stop smaller operations from benefiting from this technology.
Open-source AI models typically have lower upfront costs, so they’re worth considering. Businesses can also partner with expert IoT and AI third-party services if they’re worried about managing the inherent complexity.
AI poses opportunities and challenges in video surveillance
AI is too useful a tool in video surveillance to overlook. At the same time, using it in these applications can introduce several major concerns, raising the need for a slower, more thoughtful approach to AI adoption.
Security operations must consider all the potential challenges of AI in video surveillance before investing in it. It’s possible to overcome these obstacles, but only by paying enough attention to each one.